All Insights Article Data Mesh: Serving Fresh And Real-Time Data, Every Single Time
Data Mesh: Serving Fresh And Real-Time Data, Every Single Time
Introduction to data mesh
Data mesh is very similar to cooking at scale (as in Indian-style weddings!), where each ingredient is sourced, sliced, stored, and provided to the chef for creating delicious dishes. The task of finding each ingredient – fruits, vegetables, dairy products, spices – is done by the most knowledgeable people for each one. Team members are given self-service tools and techniques for prepping the ingredients. Finally, the whole process is governed by a set of global standards (i.e., procuring only from top vendors) and local standards (i.e., defining the right temperature). This decentralized approach ensures that every dish is consistent in taste and served at the right time.
Data mesh is a people-, process-, and technology-focused approach to building a decentralized analytical data architecture. It leverages a domain-oriented, self-service design and shifts the responsibilities for specific data sets to the people closest to that data. While the governance remains with a primary team, the responsibility for providing and managing analytical data shifts to the domain groups, supported by a common data platform team.
As agility, intelligence, automation, and real-time decision-making become critical pillars for businesses, data mesh is a great tool to have in your toolbox.
The evolution and the inflection point
Let’s take a walk through the evolution of analytical data architectures. It all started in the 1960s when data warehouses and data marts were introduced as the solution to the analytical needs of organizations. As technology progressed and computing became more economical, the architecture shifted toward the present data lake and cloud-based data fabric solutions [Figure 1]. Analytical capabilities grew with each iteration of the architecture—from simple SQL-based analysis to more visual and advanced artificial intelligence/machine learning (AI/ML) predictive analytics.
Figure 1: Evolution of Analytical Data Architecture (1960 – Present)
Despite architectural advancements, the core assumptions of the solutions around monolithic and technology-driven architecture have been largely unchallenged. At the proverbial ten-thousand-foot view, the data platform architecture resembles Figure 2, an imposing architecture that aims to source data from everywhere, cleaning, enriching, and serving it to various consumers with diverse needs. This massive task is compounded by the central team of data and ML engineers who have limited domain and business understanding but are responsible for meeting consumption needs. For instance, consider a pharmaceutical company that launches a new “buy-and-bill” drug. Introducing just one new key performance indicator (KPI), such as “conversion rate” (from prescription to administration), requires the ingestion of new data, cleansing, preparation, and aggregation. The components of the whole pipeline must be changed, limiting the ability to achieve higher velocity for large-scale and complex analysis. This situation creates an inflection point where results are no longer proportional to the investments.
Figure 2: Monolithic Analytical Data Architecture
A 2022 McKinsey survey showed that high performers engage in “frontier” practices more often, enabling AI development and deployment at scale, or what some call the “industrialization of AI.” For example, leaders are more likely to have a data architecture that is modular enough to accommodate new AI applications rapidly.1
The practical data mesh
To build a data mesh that is both functional and efficient, follow these fundamental concepts:
-
Identify business ownership: Identify the various business domains within the organization and determine the data requirements for each. This step involves working with business leaders to understand their data needs and the business outcomes they are trying to achieve.
-
Create self-organizing teams: Each team should have ownership and control over its own data domain and be responsible for its quality, governance, and management.
-
Implement data infrastructure: Implement the infrastructure required to support the data mesh approach, including setting up data platforms, data governance frameworks, and data quality standards. Design the infrastructure to support the needs of each self-organizing team and allow for easy information sharing across them.
-
Define data as a product: Data products are the deliverables of a data mesh approach, and each team should define, create, and manage these products based on specific analytical and operational needs of their domain.
-
Establish data contracts: The self-organizing teams should define their expectations for each other by establishing data contracts, thus ensuring that each team consistently and reliably meets their data consumers' quality standards and requirements.
-
Federated governance model: This principle ensures that standard policies and guidelines encourage interoperability between data products. The main goal is to ensure adherence to organizational rules, privacy, compliance, and security guidelines.
-
Monitor and optimize: Continuously monitor the data mesh solution to ensure it meets the organization’s needs, and optimize the solution when necessary to ensure it provides value and delivers business outcomes.
Expert tips for implementing data mesh
In addition to the core framework described above, the following best practices will ensure the successful implementation of a data mesh:
Implement individual models based on data warehouse modeling techniques in each bounded context. Next, have the platform solution team standardize the language in which different contexts interact. For best results, use the “customer-supplier” pattern wherein upstream and downstream teams collaborate so each can succeed independently when defining the contracts between domains.
When “defining data as a product,” remember that this requires critical mindset changes, such as measuring success through adoption, satisfaction, and establishing trust.
Finally, your central governance team should include representatives from different stakeholder groups. How these groups define your global policies on interoperability, collaboration, security and access, privacy, compliance, and documentation is critical for the success of a data mesh.
Domain-driven design (DDD) should be the core of data mesh architecture. Using the concept of bounded context, the basic premise of DDD is that a business function, like customer relationship management (CRM), can be modeled differently depending on the context. Context mapping or “data contracts” define the relationships and integrations between different contexts.
Applying a DDD results in domain-oriented analytical data, which can be defined by three archetypes:
-
Source-aligned domain data product – reflects business facts close to the origination system, for example, Veeva CRM data.
-
Aggregated domain data product – provides an integrated and aggregated view of multiple source-aligned domain data. For example, sales and activity analysis requires gathering and aggregating data from numerous source-aligned domains like CRM, sales, customer, product, etc.
-
Consumer-aligned domain data product – ensures fit-for-purpose domain data for a particular use case. For example, next best action (NBA) is a machine learning model that analyzes past touchpoints, both personal and non-personal, and provides recommendations for the next action. However, to perform the analysis, the engine requires data from multiple source-aligned domains such as CRM, sales, finance, etc. Then it transforms the source domains into a more fit-for-purpose environment.
At Axtria, we integrate data mesh thinking and processes in our product, Axtria DataMAx™, to help our customers close the gap between how data is produced and how it is consumed. We move away from point-to-point extract, transform, and load pipelines, increasing trust and adoption while driving efficiency and bringing significant productivity gains.
The figure below shows a reference architecture for implementing data mesh:
Figure 3: Data Mesh Conceptual Reference Architecture
Conclusion
In conclusion, data mesh can make organizations more agile during their analytics-at-scale journey.
We have outlined critical directional guidance for organizations seeking to embark on the data mesh journey. This effort can be costly and brings challenges across cultural shifts, change management, governance, skills, and data accessibility. By getting the fundamentals right early on, you will increase your chances of finding success.
References
- Mayhew H. Capabilities. McKinsey. December 6, 2022. Accessed August 24, 2023. https://www.mckinsey.com/capabilities/quantumblack/our-insights/the-state-of-ai-in-2022-and-a-half-decade-in-review
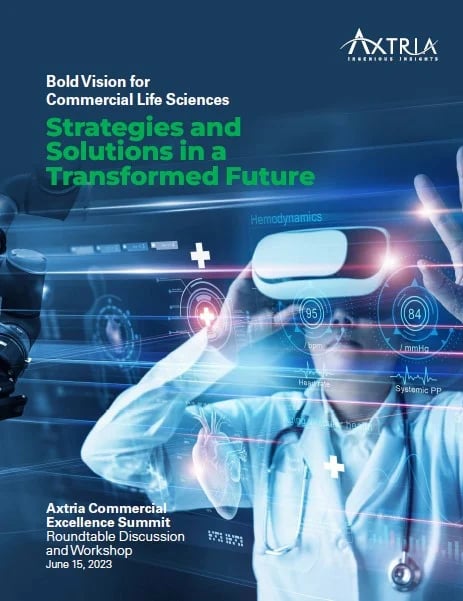
Complete the brief form to download the white paper
Related insights
Stay current on topics you care about
Recommended insights
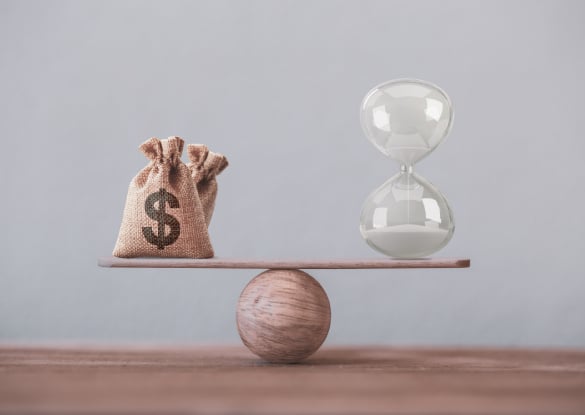
Article
Balancing Effort Versus Value: Why On-Demand Marketing Mix Modeling Software Is Essential for Brand Success
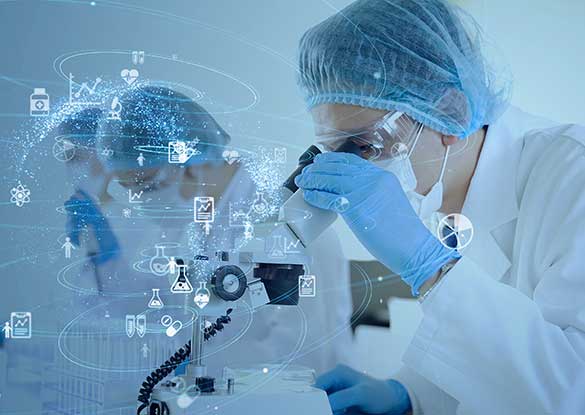
Blog