All Insights Article Rescuing Clinical Study Reports: 30% Faster Authoring With Generative AI
Rescuing Clinical Study Reports: 30% Faster Authoring With Generative AI
The ability to efficiently and accurately document clinical trial outcomes is critical to a pharmaceutical product’s approval and launch. Central to this process is the creation of Clinical Study Reports (CSRs). A CSR is a detailed document that summarizes the methods and results of a clinical trial, providing a comprehensive record of the trial. Their primary objective is to inform regulatory agencies about the safety and effectiveness of a new treatment. This information is critical for deciding whether to approve the therapy for public use. CSRs include details about the study’s design, the participants involved, the interventions used, the data collected, and how that data was analyzed. They also discuss any safety issues that arose during the trial. CSRs are critical in the decision-making that ultimately impacts patient care and drug approval.
International regulatory guidelines, such as ICH E3, mandate that CSRs be formatted consistently and contain all necessary information. Creating these reports to meet regulatory standards has always been labor-intensive. It demands the collaborative effort of medical writers, disease subject matter experts, and biostatisticians, who meticulously analyze and compile extensive clinical data. This conventional approach, though thorough, is fraught with challenges, including high costs, significant time investments, and the potential for human error. The variability in writing styles among authors can also lead to inconsistencies in report quality.
ICH E3 CSR stands for International Conference on Harmonisation of Technical Requirements for Registration of Pharmaceuticals for Human Use, Guideline E3 for Clinical Study Reports. The ICH E3 CSR is a guidance document that describes the format and content of a CSR that will be acceptable to all regulatory authorities in the ICH regions.1
As pharmaceutical companies seek to enhance efficiency and maintain high standards of accuracy, innovative solutions are being explored. Generative AI (GenAI) is a promising approach to streamline CSR authoring. By leveraging advanced natural language processing (NLP) capabilities, GenAI demonstrates the possibility of simplifying the creation of these critical documents, addressing many of the challenges inherent in the traditional approach.
In this article, we delve into the transformative potential of GenAI in the context of CSR authoring. This article will explore how this technology can accelerate the documentation process and enhance the quality and consistency of CSRs.
What Is the CSR Authoring Process, and Why Is Automation Needed?
Traditionally, CSR authoring involves meticulous efforts from teams of medical writers, disease subject matter experts, and biostatisticians who sift through vast amounts of clinical trial data to synthesize comprehensive reports. It happens through a step-by-step process:
-
The CSR process begins with the finalization of the protocol and Investigator Brochure (IB), including the preparation of a list of Institutional Ethics Committees (IECs), Institutional Review Boards (IRBs), and Principal Investigators (PIs), as well as the development of the Case Report Form (CRF).
-
Following this, patient enrollment, their randomization, and data collection take place, encompassing the creation of the Statistical Analysis Plan (SAP), documentation of any protocol deviations, and capturing of Adverse Events (AEs) and Serious Adverse Events (SAEs). Clinical trial data is collected, validated, and reconciled throughout the clinical trial until database lock is achieved.
-
The next phase involves generating statistical results, which includes the creation of Study Data Tabulation Model (SDTM) datasets, Analysis Data Model (ADaM) datasets, and the compilation of Tables, Listings, and Figures (TLFs) to analyze the clinical trial results.
-
The Clinical Study Report (CSR) is generated through drafting, review, and approval stages, culminating in the finalization of the CSR.
-
The process concludes with the submission of the finalized CSR to regulatory authorities.
The CSR authoring process faces several significant challenges that can impact efficiency, accuracy, and overall quality:
-
Cost and time constraints: Manual CSR creation demands substantial financial resources and time due to the involvement of skilled professionals such as medical writers, disease subject matter experts, and biostatisticians over prolonged periods. This process often involves multiple rounds of data collection, analysis, and review, making it both time-consuming and expensive.
-
Coordination and collaboration: The traditional process requires seamless coordination and cooperation between various stakeholders, including medical writers, statisticians, and clinical researchers. Managing this collaborative effort is challenging, particularly in geographically dispersed teams.
-
Data management challenges: Managing and integrating large volumes of clinical trial data from various sources can be complex and error-prone. Ensuring data integrity, consistency, and accessibility throughout the CSR authoring process is critical but challenging, especially when dealing with heterogeneous data formats and sources.
-
Possibility of human error: Despite rigorous quality checks, manual authoring can result in inadvertent errors and discrepancies in interpretation, potentially impacting the report’s overall quality. Human errors can occur at various stages, from data entry and analysis to report writing and editing, leading to inaccuracies affecting regulatory submissions and decision-making.
-
Inconsistent quality: Individual writing styles can introduce inconsistency and subjectivity, making the CSR generation dependent on specific authors. This variability can result in differences in tone, structure, and detail across different sections of the report, potentially compromising the overall coherence and professionalism of the document.
-
Regulatory compliance: Adhering to regulatory requirements and guidelines (e.g., ICH E3) is essential for CSR authoring. Ensuring compliance involves meticulous attention to detail and a thorough understanding of regulatory standards, which can be daunting and time-intensive.
-
Review and approval bottlenecks: Multiple rounds of review and approval are necessary to ensure the accuracy and quality of the CSR. This iterative process can create bottlenecks, delaying the completion of the report and subsequent regulatory submissions.
These challenges call for an intelligent solution to make the CSR authoring process faster, better, and cheaper while maintaining high standards of quality and coherence. GenAI offers a transformative approach to address these challenges by automating and enhancing the efficiency of CSR creation, ensuring consistency, accuracy, and regulatory compliance.
How Can GenAI Optimize the Process of Authoring CSRs?
GenAI offers a transformative solution to streamline CSR authoring. By leveraging advanced NLP capabilities, GenAI can enhance efficiency in tedious and error-prone tasks. These include accurately interpreting clinical summary tables and graphs, analyzing long clinical trial documents such as protocols and annotated case report forms (CRFs), and generating the first draft of CSR documents. This significantly reduces the time and resources required for manual authoring, expediting the generation of CSR reports and enabling quicker decision-making and regulatory submissions.
Let’s look at an example of a CSR “Adverse Event” summary. Figure 1 illustrates how summaries are written in CSRs, and Figure 2 illustrates a GenAI-generated summary based on the input table. The GenAI output includes a more comprehensive overview of the input table and is confined to the mandatory regulatory standards. These examples serve as compelling illustrations of the capabilities of GenAI in accurately summarizing input tables to elucidate critical findings.
Table 1: Illustrative input table for generating an adverse event summary for a CSR
Figure 1: Illustrative CSR text
Figure 2: GenAI-generated text. The green text represents acceptable sentence formation and matches the CSR text closely
A GenAI solution to streamline the CSR authoring process includes the following elements:
-
Scope identification: Before developing a GenAI model, defining the scope of automation is crucial. The CSR template sections are drafted using various data sources. For example, when drafting a section on “Safety Evaluation,” Adverse Events TLFs are some of the many input files.
Figure 3: Illustrative list of input data sources to ICH E3 CSR template sections -
TLF pre-processing to create a GenAI-ready dataset (GRD): Before feeding the selected TLFs to the GenAI model, pre-processing ensures the model can read the TLFs correctly and produce the needed content. This involves developing prompts to guide the model in generating accurate and scientifically precise summaries.
-
GenAI output validation: Output validation is crucial to ensure the generated content meets the desired standards and aligns with the intended purpose. Criteria for validation include accurate reasoning, no hallucinations, scientific tone, completeness, and reproducibility. An evaluation framework helps ensure the quality of generated narratives, focusing on numerical consistency, completeness, and the use of scientific language.
-
Fine-tuning the output: GenAI models involve adjusting their parameters to adapt to a specific task or dataset. Iterative refinement and regular feedback from expert medical writers improve content quality.
What Are the Potential Benefits of Using GenAI to Author CSRs?
-
Enhanced efficiency and speed
-
GenAI can process vast amounts of data and generate initial CSR drafts significantly faster than traditional methods. Axtria’s thorough examination of this efficiency translates to at least a 30% reduction in overall CSR generation time.
-
GenAI analyzes complex datasets and synthesizes information into well-structured reports, enhancing the overall quality and consistency of CSRs.
-
Automating repetitive tasks frees researchers and clinical teams to dedicate their time to higher-value activities, such as study design and analysis.
-
-
Faster regulatory submissions: Reduced CSR generation time and fewer iterations and review cycles can significantly accelerate the process of submitting clinical trial data to regulatory agencies.
-
Cost-effectiveness: Increased automation through GenAI minimizes costs by optimizing resource allocation throughout the CSR creation process.
-
Versatility beyond CSRs: The same GenAI technology can be adapted to generate other crucial clinical trial documents, including patient narratives, drug safety reports, clinical data management plans, and data transfer agreements.
Despite challenges such as data privacy concerns, domain-specific knowledge gaps, and the need for interpretability, the potential benefits of GenAI for CSR authoring are undeniable. Addressing these challenges through robust data governance frameworks, upskilling personnel, and stringent validation processes can unlock new levels of efficiency and accuracy. Collaboration between domain experts, data scientists, and regulatory bodies will be critical in ensuring the ethical and responsible use of GenAI in clinical settings. By embracing innovation while maintaining high patient safety standards, data integrity, and regulatory compliance, GenAI can encourage transformative advancements in clinical research.
References
- ICH.org. Accessed June 4, 2024. https://www.ich.org/page/efficacy-guidelines
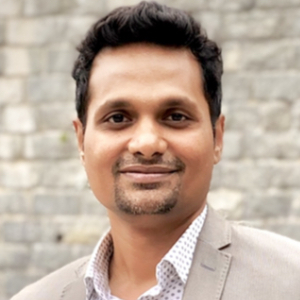
Shekhar Thumake
With 18 years of industry experience, including over 16 years in clinical development, Dr Shekhar Thumake serves as the Director of Clinical Solutions at Axtria. He has a solid clinical foundation complemented by a MBA in general management, specialization in data science, business analysis, and big data analytics. In his current role, he spearheads the offshore delivery of clinical information management and clinical data science services, leveraging cutting-edge technologies. Dr Shekhar excels in creating innovative solutions using advanced AI, machine learning (ML), and generative AI (GenAI). His work focuses on enhancing the efficiency of clinical development processes and making new therapeutics more affordable and accessible. With a deep interest in GenAI, natural language processing (NLP), large language models (LLM), deep learning, and traditional statistical modeling, he is committed to transforming clinical development through the power of modern data science and analytics.
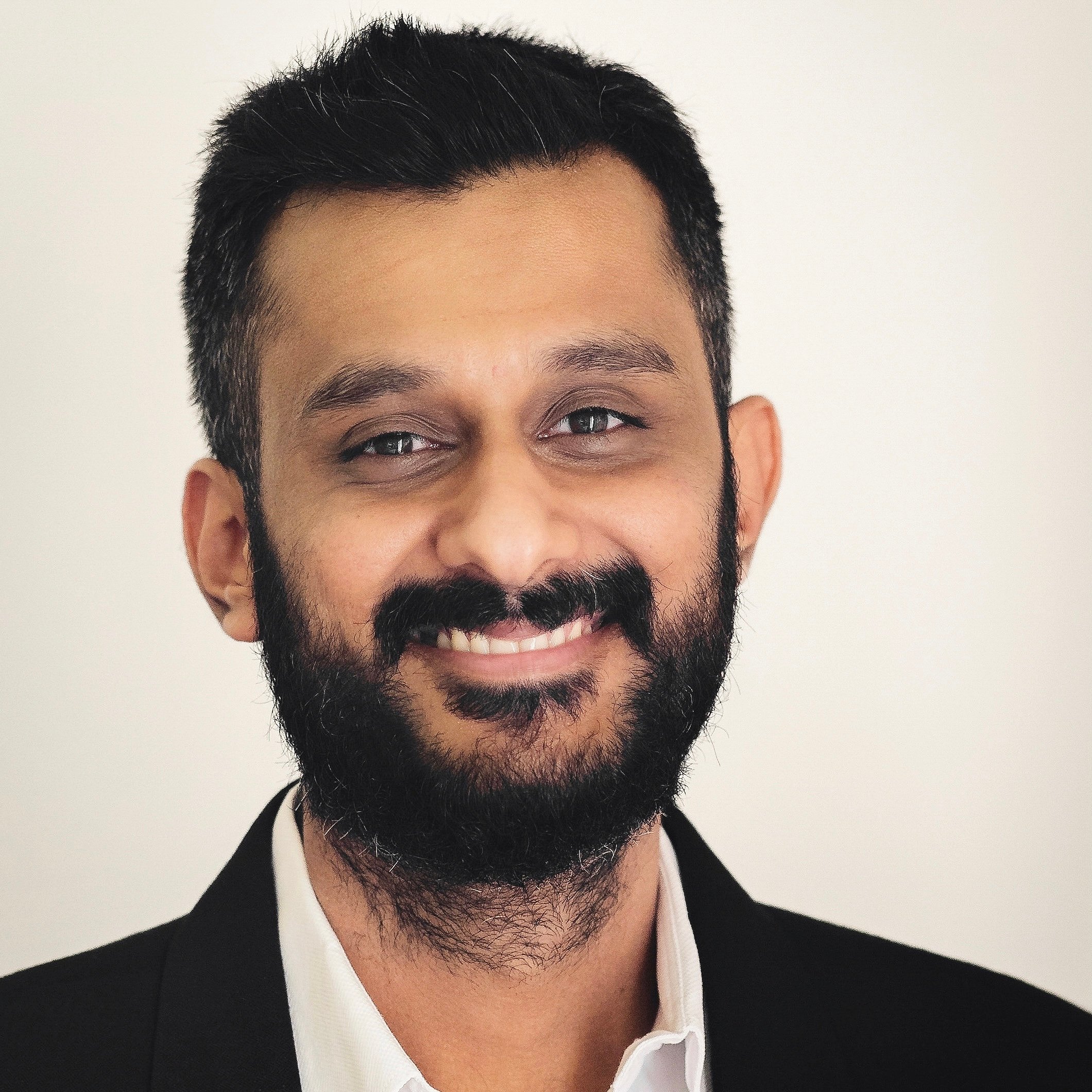
Suraj Gupta
Suraj Gupta is a Senior Manager, Marketing at Axtria. He has over 9 years of experience in the analytics and consulting industry, with more than 6 years with life sciences. At Axtria, Suraj has contributed to sales and marketing functions, including business and client development, knowledge management, digital marketing, business intelligence, and content writing. Suraj has an MBA degree in Marketing and a graduate degree in Economics.
Complete the brief form to download the white paper
Related insights
Decoding Data Democratization
Stay current on topics you care about
Recommended insights
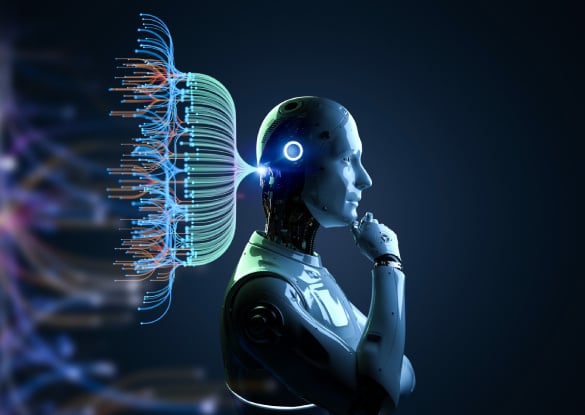
Article