All Insights Article Revolutionizing Evidence Synthesis With AI: Insights From NICE's Latest Position Statement
Revolutionizing Evidence Synthesis With AI: Insights From NICE's Latest Position Statement
Revolutionizing Evidence Synthesis With AI: Insights From NICE's Latest Position Statement
Discover the transformative potential of AI across systematic reviews, clinical evidence, real-world data, and cost-effectiveness models, while ensuring ethical responsibility in health technology assessments.
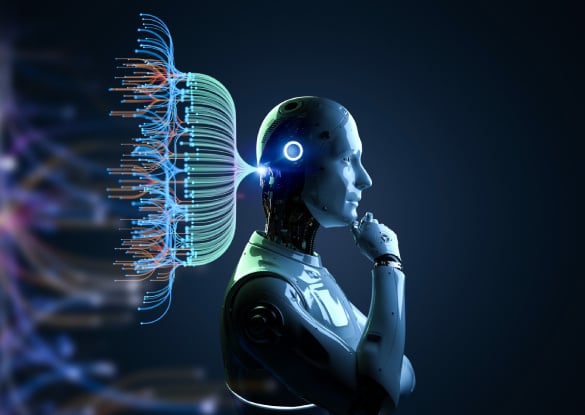
In an era where data is abundant but actionable insights are scarce, the National Institute for Health and Care Excellence (NICE) has taken a pioneering step by releasing a position statement on the use of artificial intelligence (AI) in evidence generation.1 This move underscores the transformative potential of AI in health technology assessments (HTAs) while emphasizing the need for ethical and transparent practices. This position statement clarifies how NICE will evaluate the use of AI methods in generating and reporting evidence within its guidance production programs. It outlines potential AI applications that are either currently used or are being researched in HTA. AI is a rapidly evolving field, and the listed uses are not exhaustive. The outlined potential uses do not imply endorsement or acceptance by NICE. Organizations considering AI methods in their evidence should discuss their plans with NICE.
The Promise of AI in Evidence Generation
AI technologies, including machine learning and generative AI, are revolutionizing the way we handle and analyze large datasets. These advanced methods can uncover patterns and relationships that might elude human analysts, thereby enhancing the quality and depth of evidence generated for health technologies. NICE recognizes this potential and anticipates a growing role for AI in evidence synthesis. This article provides a brief overview of areas within HTA where AI methods can be deployed (Figure 1).
Figure 1: The Promise of AI in Evidence Generation
Systematic Review and Evidence Synthesis
Traditional literature search and review processes are manual and resource-intensive. AI methods can automate many steps, including generating search strategies, classifying studies, screening records, and visualizing results. Although these applications are still developing, large language models can also automate data extraction and generate code for meta-analyses. Cochrane2 and the Guidelines International Network3 are creating guidance on the responsible use of AI in evidence synthesis, which will be valuable for organizations adopting these methods.
Clinical Evidence
Clinical evidence is derived from clinical trials and real-world data, quantifying treatment effects, side effects, and generalizability to the National Health Service (NHS) population. AI can enhance trial design by defining criteria, optimizing dosage, sample size, and duration, and using natural language processing (NLP) to mine health records for suitable participants and side effect reporting. AI can also address data limitations by identifying relevant covariates, reducing bias, improving prediction accuracy, and modeling complex relationships. It can predict effectiveness in different populations and generate synthetic data and external control arms, especially when placebo use is unethical. Natural language processing can analyze large datasets to create executive summaries and simplify technical language for lay summaries. Transparency in AI use is crucial, with relevant checklists like PALISADE (Purpose, Appropriateness, Limitations, Implementation, Sensitivity and specificity, Algorithm characteristics, Data characteristics, Explainability)4 and TRIPOD-AI (Transparent Reporting of a multivariable prediction model for Individual Prognosis Or Diagnosis- Artificial Intelligence) ensuring rigorous reporting and justification of AI methods in clinical trials.
Real-World Data and Analysis
As large datasets reflecting routine care and real-world populations become more accessible and standardized, AI methods are increasingly valuable in generating real-world evidence. AI can automate data processing tasks such as transforming unstructured data into structured formats, integrating multiple data sources, and improving data quality through matching, linkage, deduplication, and error detection.
AI also aids in selecting relevant populations and observations from extensive datasets to address specific research questions. Additionally, AI methods can estimate comparative treatment effects by selecting relevant features for model construction and providing targeted causal effect estimates using multiple machine learning algorithms.
Cost-Effectiveness Evidence
Cost-effectiveness evidence is typically derived from economic models that involve a multi-step, resource-intensive process. AI methods can enhance several steps, including model conceptualization, parameter estimation, construction, validation, analysis, and reporting. AI can also analyze complex datasets to uncover new insights into cost drivers and health outcomes, informing the conceptualization and parameterization of economic models. Large language models can automate economic models' construction, calibration, and reporting, allowing for the comparison of multiple models to assess structural uncertainty.
These models can also be updated with the latest information, such as clinical data or comparators, facilitating real-time updates. AI methods can support the replication and cross-validation of existing models, ensuring robustness. Machine learning can optimize simulation processes, reducing computational time and making more complex models practical for use, including in probabilistic sensitivity analysis.
Key Points to Consider
Balancing Innovation with Caution
While the benefits of AI are clear, NICE’s guidance is rooted in a cautious approach. The organization stresses that AI should only be employed when it offers demonstrable value, meaning rigorous validation processes are essential to mitigate risks such as algorithmic bias and cybersecurity threats. Transparency is paramount, with NICE requiring detailed documentation of AI methods, their rationale, and the steps taken to address potential risks (Figure 2).
Figure 2: Key Points for AI Implementation
Adherence to Standards and Guidelines
NICE’s position statement emphasizes the importance of adhering to established regulations, good practices, and standards. These frameworks ensure that the evidence produced is accurate, reliable, and ethically sound. By adhering to these standards, organizations can maintain the integrity of their findings and build trust in their AI-driven processes.
The Role of Human Oversight
A core tenet of NICE’s guidance is the principle of human oversight. AI should augment, not replace, human judgment. This approach ensures that a capable and informed human remains in the loop, maintaining trust in AI’s contributions to HTAs. Human oversight is crucial for interpreting AI-generated insights and making informed decisions that align with broader ethical frameworks.Building a Transparent and Trustworthy AI Ecosystem
Transparency is a cornerstone of NICE’s guidance. Organizations must be open about the way they use AI, providing clear justifications for their methods and outlining the assumptions made. This transparency helps mitigate risks and ensures that AI applications do not compromise the quality or integrity of the evidence produced. NICE aims to balance innovation with ethical responsibility by fostering a transparent and trustworthy AI ecosystem.
Next Steps and Future Directions
NICE will reassess this position statement if new, significant evidence necessitates changes to AI methods for evidence submissions. Potential updates to the health technology evaluation manual will be considered within the framework for modular updates. NICE will monitor the use of AI in evaluations, assessing challenges and opportunities, and will continue to evaluate the need for upskilling staff and committee members. Future efforts may include expanding capacity in disciplines supporting AI adoption. NICE will maintain its policy and research activities on AI methods in HTA.
Conclusion
The guidance provided by NICE for the use of AI in evidence generation marks a significant milestone in the integration of advanced technologies into HTAs. By emphasizing transparency, rigorous validation, and human oversight, NICE is paving the way for a future where AI can enhance evidence synthesis while upholding the highest standards of ethical and regulatory compliance.
Axtria is at the forefront of using advanced analytics and AI methods across the RWE, HEOR, and evidence synthesis spectrum. For example, Axtria's recent whitepaper on the Use of Natural Language Processing in Literature Reviews highlights that by utilizing natural language processing, researchers can cut the time needed for literature reviews by 50%, allowing them to focus more on in-depth review and analysis. This white paper demonstrates how contemporary data scientists are merging human precision with the speed of AI.
In summary, NICE’s vision for AI in evidence synthesis holds cautious optimism, where innovation is in balance with ethical responsibility, and human judgment remains central to the decision-making process. This guidance sets the stage for a future where AI can truly transform the landscape of health technology assessments, making them more efficient, accurate, and impactful.
References
- National Institute for Health and Care Excellence. Use of AI in evidence generation: NICE position statement. 2024. Accessed September 10, 2024. https://www.nice.org.uk/about/what-we-do/our-research-work/use-of-ai-in-evidence-generation–nice-position-statement
- Noel-Storr A, Flemyng E, Thomas J. Artificial intelligence (AI) technologies in Cochrane. May 9, 2024. Accessed September 10, 2024. https://training.cochrane.org/resource/artificial-intelligence-technologies-in-cochrane
- Guidelines International Network (GIN, 2024) Working groups: artificial intelligence. Accessed September 10, 2024. https://g-i-n.net/get-involved/working-groups
- Padula WV, Kreif N, Vannss D J, Adamson B, Rueda J D, Felizzi F, Johsson P, Ijzerman M J, Butte A, Crown W. Machine Learning methods in health economics and outcomes research—the PALISADE checklist: a good practices report of an ISPOR task force. Value Health. 2022; 25(7):1063-1080. doi: 10.1016/j.jval.2022.03.022.
- Collins GS, Moons KGM, Dhiman P, Riley R D, Beam A L, Van Calster B, Ghassemi M, Liu X, Reitsma J B, van Smeden M, Boulesteix A - L, Camaradou J C, Celi L A, Denaxas S, Denniston A K, Glocker B, Golub R M, Harvey H, Heinze G, Hoffman M M, Kengne A P, Lam E, Lee N, Loder E W, Maier-Hein L, Mateen B A, McCradden M D, Oakden-Rayner L, Ordish J, Parnell R, Rose S, Singh L, Wynants L, Logullo P. (2024) TRIPOD+AI statement: updated guidance for reporting clinical prediction models that use regression or machine learning methods. BMJ 2024; 385:e078378. https://www.bmj.com/content/385/bmj-2023-078378
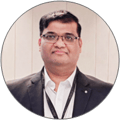
Javed Shaikh
Javed is a Director in RWE, HEOR & Evidence Synthesis practice, managing delivery and governance of several global accounts across US & EU. With over 16 years of expertise, he excels in generating and leveraging evidence to craft unique and persuasive value propositions throughout the product lifecycle, ultimately facilitating patient access to innovative treatments.
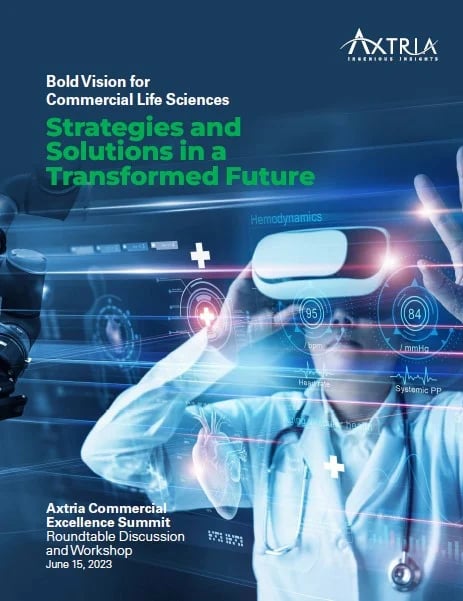