All Insights Article Unfolding The Data Lakehouse: Far-Reaching And Forward-Looking!
Unfolding The Data Lakehouse: Far-Reaching And Forward-Looking!
Unfolding The Data Lakehouse: Far-Reaching And Forward-Looking!
The adoption of data lakehouse frameworks will increase in the near future as they completely remove the issue of data swamps. That, in turn, will enable transparent, easy-to-adopt, cost-efficient systems for data management.
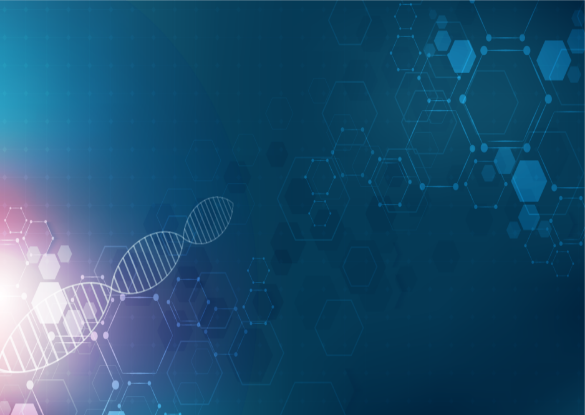
Right now, life sciences companies have a pressing need: a flexible and high-performance data processing and analysis system that can handle diverse data applications such as SQL analytics, real-time monitoring, machine learning (ML), and artificial intelligence (AI). The data for such analysis is primarily unstructured and includes a variety of file types like raw data, images, video, audio, and text. This diverse information is a challenge for any data management system. Therefore, traditional enterprise data architectures include multiple systems to handle the complexity. The integrated ecosystem includes a data lake, several data warehouses (DW) for different business groups, streaming APIs, image databases, and more. Such ecosystems tend to be complex in design and expensive to maintain.
Enterprise data management systems designed with DWs and data lakes are experiencing challenges. The volume of data is growing so quickly that it is difficult to incorporate it into unified analytical platforms. Even minor errors in this step can create gaps that lead to incorrect messaging and insights. Any delay in ingesting the data, analyzing it, and drawing insights from it can affect the time to market and business decisions for things like territory alignment. While DWs offer tremendous advantages like data standardization, quality, and consistency, they lack flexibility and incur high maintenance costs. Data lakes, on the other hand, provide greater flexibility to data scientists and can support a wide variety of use cases but falter when it comes to data security and robustness. With the increase in the data volume, data lakes often become data swamps of disorganized data. Fortunately, the data lakehouse system has evolved to overcome these challenges.
Data Lakehouse Architecture Explained
This new, open data management architecture adopts the best of both worlds to combine a data lake's flexibility, cost-effectiveness, and scale with the management features, speed, and robustness of a DW. It allows atomicity, consistency, isolation, and durability in performing data transactions (ACID transactions).
Figure 1: Data Lakehouse Architecture
The data lakehouse architecture above applies the DW’s metadata layer onto the raw data stored in the data lake. Data lakehouses also provide features that lead to better data management, query optimization, and performance improvement, such as:
Better business intelligence (BI) and visualization:
-
Direct interaction of various BI tools with the data in the lakehouse eliminates the need to maintain duplicate copies of the data.
-
Data is available in near-real-time, with very little latency.
-
The timely reporting and faster analytics of data lakehouses make it possible to generate better insights.
ACID transaction support:
-
Enables ACID transactions for the data lake and ensures consistency as multiple parties concurrently read or write data, typically using SQL.
-
Operations such as MERGE, which can be executed directly on datasets.
-
Audit history, which can easily be maintained using Time Travel features.
Better data governance:
-
Lakehouses support schema validation that ensures data quality by rejecting writes to a table that does not match the table’s schema.
-
Robust governance and auditing mechanisms in data lakehouses allow greater control over security, access, metrics, and other critical data management aspects.
Unstructured data support:
-
Real-time reports and support for streaming
-
Provides APIs for a variety of tools; allows access to the data by engines such as ML, AI systems, and R/Python libraries
-
Ability to store, refine, analyze, and access the data types needed for many new data applications, including images, video, audio, semi-structured data, and text
Best practices for data lakehouse implementations
While implementing data lakehouses, the following best practices must be kept in mind:
The data lakehouse should act as a landing zone for all data: It is recommended that transformations on raw data must not be performed in the data lakehouse unless it is personally identifiable information. It is best to save data in its native format.
Data lakehouses provide role and view-based access: Setting up role-based access is a good starting point but not enough. View-based access control allows precise slicing of permission boundaries down to row and column levels using SQL views.
Catalog the data in the data lakehouse: Catalog the new data entering the data lakehouse and continually curate it to ensure it remains updated. This catalog is an organized, comprehensive store of table metadata, including table and column descriptions, schema, data lineage information, etc.
Conclusion
Data lakehouses present an exciting new horizon in next-generation data management solutions. Their ability to use unstructured data with AI, ML, and automated data initiatives can bring value to organizations. The adoption of data lakehouse frameworks will increase in the near future as they completely remove the issue of data swamps. That, in turn, will enable transparent, easy-to-adopt, cost-efficient systems for data management.
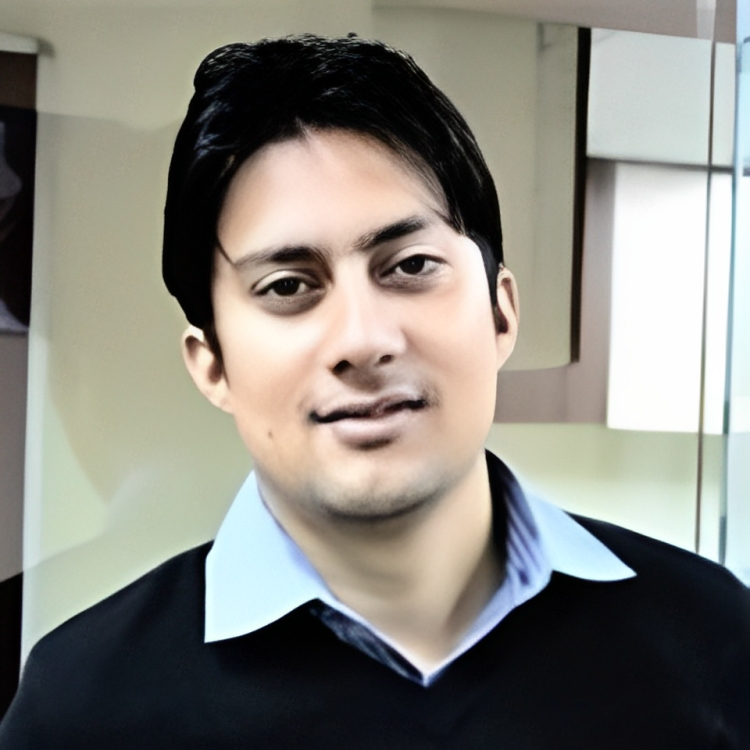
Rajeev Bhardwaj
Rajeev is a Solutions Architect with the Axtria Technology CoE team and has expertise in big data engineering, business information management, cloud computing, and advanced analytics.
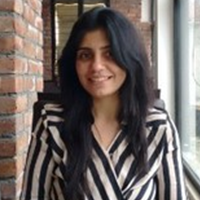
Megha Kapadia
Megha is a B2B marketing strategist focusing on thought leadership content and brand messaging. She conducts growth marketing initiatives right from content planning, creation, contact and account mining, and customer segmentation to driving social media and email campaigns to generate business leads.
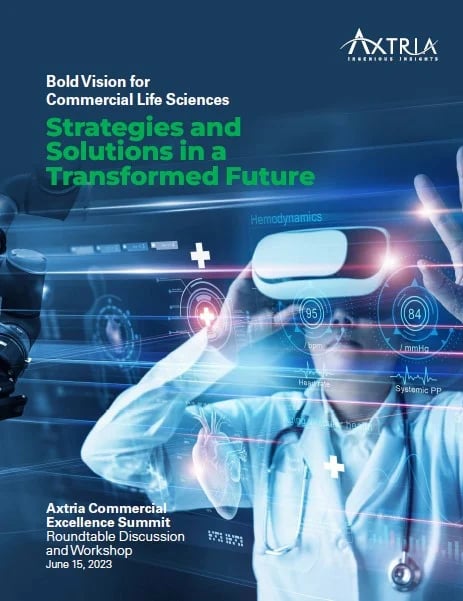
Complete the brief form to download the white paper
Related insights
Decoding Data Democratization
Data Management: What It Is and Why It’s Important
Stay current on topics you care about
Recommended insights
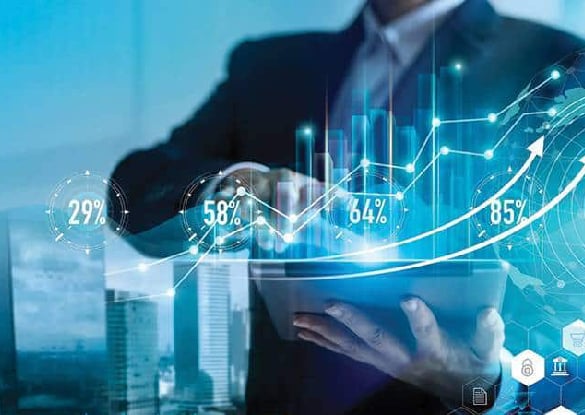
Article