All Insights Article Building Robust, Generative AI-Ready Datasets
Building Robust, Generative AI-Ready Datasets
Introduction
We are witnessing a revolution in pharmaceutical and life sciences data management. Every company is angling to include generative artificial intelligence in their workflow. Introducing natural language interactions provides colossal potential, from blazingly fast data retrieval to laser-like focus on a topic to immense time saved. In fact, the upside is limited only by your creativity.
But simply adding a fancy chatbot to your framework won’t cut it. None of these benefits are possible if your information assets aren’t first prepared. You need generative AI-ready data. This article explains what generative AI-ready data is and why it is the key to harnessing the true power of this revolution.
Large Language Models Have Problems with Existing Data Ecosystems
Using an existing data ecosystem for large language models (LLMs) presents a multitude of challenges. Firstly, data heterogeneity poses a significant obstacle as LLMs require diverse and comprehensive datasets, making it difficult to curate and integrate information from various sources. Secondly, data privacy and ethics concerns must be addressed to ensure the responsible use of sensitive information. LLMs are prone to inheriting biases from training data, raising issues of fairness and equity. Data quality is another pressing issue, as errors and inaccuracies in training data can lead to unreliable model outputs.
Additionally, LLMs must be adaptable to evolving data landscapes, requiring continuous updates and fine-tuning. Data rights and licensing issues can hinder access to essential datasets, and scalability challenges arise when handling vast data for training. Tackling these challenges is crucial for the responsible and effective deployment of LLMs in various applications.
Figure 1: The problems that arise when applying LLMs to existing data ecosystems.
Addressing these challenges involves a combination of data curation, preprocessing, fine-tuning, ongoing monitoring, and staying up to date on the latest regulations and compliance standards. Moreover, it's important to continuously update and adapt the LLMs to ensure they remain useful and aligned with user expectations in a changing data landscape.
The Current State of Pharma Information Management
Life sciences organizations generate a significant amount of structured data due to the nature of their operations, interactions, and syndicated data sources. This data, usually confined to tables and databases, is already organized, making it suitable for analysis and decision-making.
Now, the value of all data has grown exponentially, driven by generative AI’s capacity to handle unstructured information like conversations, videos, and code. This marks a substantial departure from the conventional landscape where organizations were primarily equipped to handle only structured information. An optimized combination of both enables a spectrum of use cases for LLMs to untap.
Figure 2: An example of a current data ecosystem in pharma.
What is a Generative AI-Ready Dataset (GRD)?
Analytics-ready datasets (ARDs), reporting-ready datasets (RRDs), and generative AI-ready datasets (GRDs) serve distinct purposes in the data processing spectrum. ARDs are meticulously prepared, having undergone extensive cleaning, transformation, and structuring to facilitate advanced data analysis and modeling. They are tailored for tasks like statistical analysis, machine learning, and data visualization, ensuring data quality and consistency. RRDs are optimized for generating regular reports and dashboards. They often have a predefined structure that aligns with specific reporting requirements, enabling efficient and consistent reporting.
In contrast, GRDs are primed to train and serve data for generative AI models, like GPT-4, and typically consist of diverse, unstructured data such as text, images, or audio along with structured data. These datasets allow generative models to learn and generate content that resembles the input data, making them valuable for applications like natural language generation and creative content creation.
Figure 3: A comparison of ARD, RRD, and GRD.
Use Cases for Generative AI-Ready Datasets
Generative AI-ready datasets have the potential to revolutionize research, accelerate drug development, and advance personalized medicine while maintaining data privacy and ethical standards in the pharmaceutical domain. There are significant benefits of GRD in the LLM lifecycle, including building custom training models, fine-tuning the pre-trained model, and context prompts enabled by information retrieval with private data.
Figure 4: Potential areas in pharma for generative AI-ready datasets.
- Optimizing HCP Medical Inquiry Responses
In responding to medical inquiries from healthcare professionals (HCPs), call centers aim to primarily utilize Standard Response Letters (SRLs) to fulfill requests, minimizing the involvement of Medical Science Liaisons (MSLs). However, there are instances when SRLs alone are insufficient, necessitating the engagement of MSLs to provide comprehensive responses. GRD-powered LLMs significantly reduce the MSL effort, resulting in a quicker turnaround and a complete response with data-driven insights. - Addressing Data Issues/Data Quality with GenAI
Frequent data issues, such as non-compliance with agreed data formats, create significant challenges in managing service providers (SPs) and service data (SDs), leading to delays in business decision-making. The complexity intensifies when dealing with many SPs and SDs in the ecosystem. A GRD-powered LLM allows faster communication with SPs while providing examples, resulting in quicker resolution and a lower negative impact on the business.
THE Future of Pharma Information Management: The Dominance of Generative AI
Harmonizing structured and unstructured (or semi-structured) data is pivotal in developing the business-centric applications we covered earlier. It involves integrating and synchronizing well-organized, structured data—typically found in databases and tables—with the more fluid and varied nature of unstructured or semi-structured data, encompassing elements like text, images, videos, and documents. By coalescing these data types, organizations can create applications that are finely attuned to their business needs and objectives. This integration process enables businesses to extract valuable insights from a wealth of data sources, enhancing decision-making processes, automating routine tasks, and improving overall operational efficiency. In essence, blending structured and unstructured or semi-structured data is the bridge that connects raw information to actionable intelligence, driving innovation and competitiveness in the business landscape.
Figure 5: An example of an LLM-enabled pharma data ecosystem, powered by GRD.
Conclusion
The need for and utilization of generative AI-ready datasets in pharma cannot be overstated. As the industry grapples with the ever-growing demand for personalized treatments and more efficient research processes, GRDs emerge as a game-changing solution. They offer a versatile means of simulating complex molecular structures, accelerating discoveries, and advancing personalized medicine, all while preserving data privacy and adhering to ethical standards. By harnessing the power of generative AI-ready datasets, pharmaceutical researchers can unlock new frontiers in drug development, ultimately leading to more effective treatments, improved patient outcomes, and a brighter future for healthcare innovation.
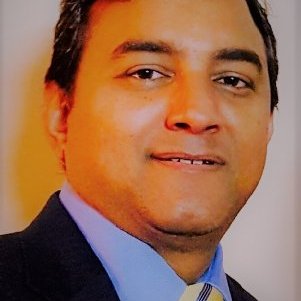
Vipin Tyagi
Vipin has 20+ years of IT experience, with more than 14 years in the Data warehousing and Business Intelligence area. Expertise includes client engagement, delivery management, project/program management, for key accounts. Proven track record in delivery of strategic programs, including architecture, design, development, implementation, integration and IT enabled transformation of enterprise application systems. He has rich experience in pharma commercial area such as Sales and Marketing, Clinical, trial operations and finance.
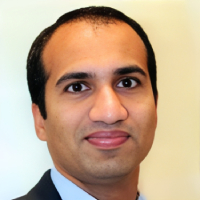
Bilahari Manipuzha
Bilahari had 15 years of experience in designing and building Data Integration and Analytic platforms for Life Science and Healthcare customers. With domain knowledge coupled with technical expertise, he has helped various global organizations to build Analytics Platform and solutions in public clouds, on-premises, and hybrid to address the business needs.
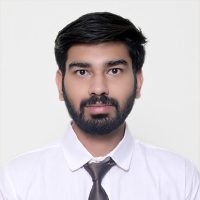
Nikhil Ojha
Nikhil is a Senior Developer with the Axtria Technology Center of Excellence team. His expertise spans Machine Learning, ML Ops, generative AI, Big Data engineering, cloud computing, and advanced analytics.
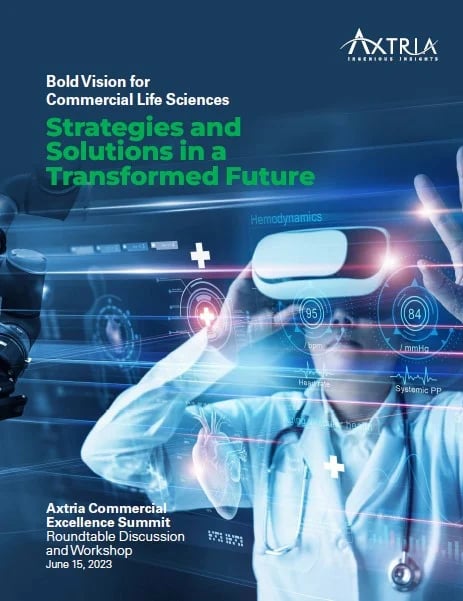
Complete the brief form to download the white paper
Related insights
Stay current on topics you care about
Recommended insights
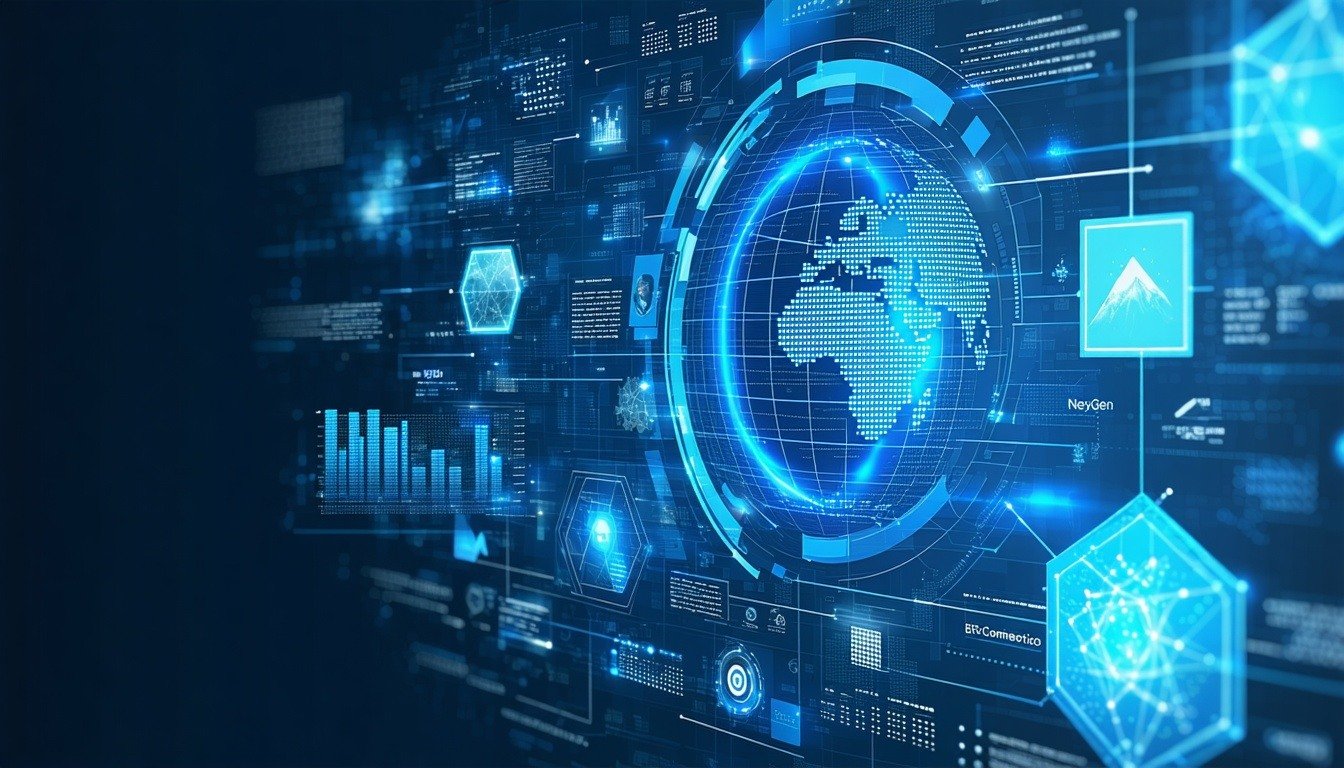
Blog