All Insights Article Generative AI: Pioneering the Next Frontier in Pharmaceutical Innovation
Generative AI: Pioneering the Next Frontier in Pharmaceutical Innovation
The pharmaceutical industry is embracing Generative AI (GenAI) as a powerful tool to revolutionize its processes. With its potential to significantly reduce the long timelines in drug development and mitigate rising costs, GenAI is providing innovative solutions to industry challenges. From accelerating drug discovery to optimizing clinical trials, the potential for GenAI to reshape pharmaceutical operations is vast. This article will explore how GenAI is transforming the pharmaceutical landscape and driving technical innovation and business growth, instilling a sense of optimism about the future of drug development.
Accelerating Drug Discovery With AI Models
Drug discovery has historically been one of the most time-intensive and expensive stages of pharmaceutical development. Usually, it takes billions of dollars and over ten years to bring a new drug to market. GenAI is poised to dramatically reduce these timelines by utilizing sophisticated machine learning models such as variational autoencoders (VAEs), generative adversarial networks (GANs), and recurrent neural networks (RNNs).
Generative adversarial networks are a creative duo of neural networks – one generates synthetic data, such as new molecular structures, while the other evaluates their quality. This pairing helps to rapidly design new drug compounds with realistic features and speed up the identification of potential candidates. Variational autoencoders take a different approach by encoding molecular data into a compressed form, allowing the generation of new data points that retain the characteristics of the original. This technique enables researchers to explore chemical properties and simulate new molecules without expensive lab experiments. Recurrent neural networks, on the other hand, excel at handling time-sequenced data, making them invaluable for predicting how drug molecules behave over time. They analyze interactions between molecules and biological systems, providing insights into a drug’s potential effectiveness and side effects early in development.
Together, these AI models can simulate molecular structures, generate new drug compounds, and predict how these compounds interact with biological systems. This way, GenAI is transforming what has historically been a lengthy and costly process into a more efficient, data-driven operation, bringing hope for faster and more affordable therapeutic breakthroughs.
Figure 1: GAN for generating drug molecules. Source: MDPI1
Platforms such as Insilico Medicine and Exscientia are leading the use of GenAI to speed up the identification of drug candidates.2 Through deep learning and predictive analytics, these platforms can screen billions of potential compounds and reduce the time spent in the initial phases of drug discovery. As a result, companies can streamline R&D efforts and create a more agile pipeline to bring innovative therapies to patients faster.
Business impact: AI-driven models significantly reduce pharmaceutical companies' R&D costs, allowing quicker decision-making and drug approvals. The real business value lies in reduced time to market, which gives companies a competitive edge when launching new products.
Personalized Medicine: AI-Driven Precision
One of the most exciting healthcare applications of GenAI is its ability to revolutionize personalized medicine. By processing vast and complex datasets, GenAI can provide a level of treatment customization that was previously unattainable. In this case, we combine transfer learning, reinforcement learning, and natural language processing (NLP).
Generative AI analyzes multimodal data in personalized medicine, including genomic sequencing, electronic health records (EHRs), and lifestyle data, to generate insights into how specific patient subgroups will respond to various treatments. Transfer learning, for example, allows pre-trained models to apply knowledge gained from one task, such as disease diagnosis, to another related task, such as treatment recommendation. Similarly, reinforcement learning models can optimize treatment plans by learning from patient outcomes, thus improving decision-making.
Additionally, by incorporating NLP techniques, GenAI can process unstructured medical records and research papers by extracting valuable insights that help formulate highly personalized treatment protocols. Pharmaceutical companies can use these insights to develop drugs that cater to specific genetic or environmental factors, ultimately leading to more effective treatments.
Technical example: DeepMind’s AlphaFold AI model, which uses deep learning to predict protein folding, is being integrated into personalized medicine research.3,4 The ability to predict protein structures based on genetic information helps us understand how drugs interact with specific proteins, which further forms the foundation for designing personalized therapies.
Business impact: Generative AI-driven personalized medicine improves patient outcomes and creates new revenue streams for pharmaceutical companies. Companies can tap into niche markets by offering highly targeted treatments that increase efficacy and customer satisfaction.
Optimizing Clinical Trials With Synthetic Data and AI Simulations
Clinical trials are an essential yet costly and time-consuming part of bringing a new drug to market. The challenges of recruiting patients, complying with regulatory standards, and ensuring safety add to the complexity. Generative AI is making clinical trials more efficient by generating synthetic patient data and creating virtual trial simulations.
Artificial intelligence models, such as generative pre-trained transformers (GPTs) and VAEs, generate synthetic data that closely mimics actual patient information and can simulate clinical trials before testing on human subjects. By running virtual trials, pharmaceutical companies can quickly assess a drug’s safety and efficacy by allowing them to narrow down the most promising candidates for real-world testing.
AI-driven patient recruitment models are also transforming clinical trials. With GenAI, it is possible to analyze demographic, genomic, and medical history data to identify the candidates most likely to respond favorably to a new drug to improve patient selection and yield more efficient trials with fewer dropouts and faster results.
Technical insight: AI models like GPT-3 generate synthetic data and automate the analysis of trial outcomes.5,6 By learning from historical clinical trial data, these models can predict potential side effects, patient outcomes, and success rates. Additionally, AI models can be trained across decentralized clinical data while preserving patient privacy with federated learning.
Business impact: For pharmaceutical companies, these innovations lead to cost reductions, faster trial completions, and fewer regulatory hurdles. Speeding up clinical trials accelerates the approval process and helps companies bring new treatments to the market sooner while ensuring safety and efficacy.
Enhancing Communication With AI-Generated Content
Because the pharmaceutical industry is so highly regulated, compliance and accuracy in communication are critical. Generative AI is transforming pharmaceutical companies’ communication with stakeholders by automating content creation, including regulatory reports, patient information leaflets, and marketing materials.
Using AI models such as GPT-3, pharma companies can quickly generate high-quality, compliant, and tailored content. These models can process structured and unstructured data and ensure that communications are timely, relevant, and follow regulatory guidelines. GenAI reduces the manual burden and speeds up workflows by generating reports for regulators or creating personalized marketing materials for healthcare professionals.
Technical insight: Natural language processing plays a significant role in automating pharmaceutical communications. Artificial intelligence-driven NLP models can extract critical data from regulatory documents and clinical trial reports to generate summaries or draft new documents. Additionally, AI ensures that content remains updated by continuously learning from new data.
Business impact: Automating content creation helps pharmaceutical companies save time and resources by ensuring faster information delivery to stakeholders, leading to better alignment with regulatory bodies and improved engagement with healthcare providers and patients..
Conclusion
Generative AI is revolutionizing the pharmaceutical industry by streamlining drug discovery, clinical trials, and personalized medicine. Advanced models like GANs, VAEs, and GPTs are at the core of this transformation.5,7,8 Generative adversarial networks accelerate drug development by generating new molecular structures, allowing for faster identification of viable drug candidates. Variational autoencoders help simulate realistic molecular data by facilitating efficient exploration of chemical properties. On the other hand, GPT models enhance decision-making by automating data analysis and predicting clinical outcomes, making research more efficient.
Pharmaceutical companies that embrace GenAI are not just gaining operational efficiencies but are redefining the boundaries of what’s possible in medicine. Whether through the development of breakthrough drugs, the optimization of clinical trials, or the personalization of therapies, GenAI is paving the way for future healthcare innovations.
References
- Park SW, Ko JS, Huh JH, Kim JC. Review on Generative Adversarial Networks: Focusing on Computer Vision and Its Applications. Electronics. 2021 May 20;10(10):1216. Available from: https://www.mdpi.com/2079-9292/10/10/1216
- Yao R. Insilico Medicine Uses Generative AI to Accelerate Drug Discovery [Internet]. NVIDIA Blog. 2023. Available from: https://blogs.nvidia.com/blog/insilico-medicine-uses-generative-ai-to-accelerate-drug-discovery/
- Callaway E. “The entire protein universe”: AI predicts shape of nearly every known protein. Nature [Internet]. 2022 Jul 28; Available from: https://www.nature.com/articles/d41586-022-02083-2
- AlphaFold: Using AI for scientific discovery [Internet]. Google DeepMind. 2022. Available from: https://deepmind.google/discover/blog/alphafold-using-ai-for-scientific-discovery/
- Generative AI in Synthetic Medical Data: A Guide [Internet]. CrossAsyst. 2024 [cited 2024 Oct 9]. Available from: https://crossasyst.com/blog/generative-ai-in-synthetic-medical-data/
- Warneck-Silvestrin L. How Is Artificial Intelligence Changing the Clinical Trials Landscape? [Internet]. Inside Precision Medicine. 2024 [cited 2024 Oct 9]. Available from: https://www.insideprecisionmedicine.com/topics/informatics/how-is-artificial-intelligence-changing-the-clinical-trials-landscape/
- Adapting LLMs to Downstream Tasks Using Federated Learning on Distributed Datasets | NVIDIA Technical Blog [Internet]. NVIDIA Technical Blog. 2023 [cited 2024 Oct 9]. Available from: https://developer.nvidia.com/blog/adapting-llms-to-downstream-tasks-using-federated-learning-on-distributed-datasets/
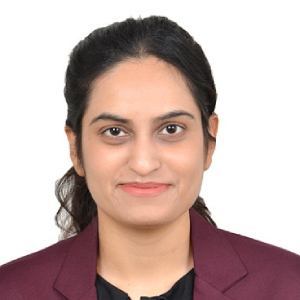
Kaveri Pradeep
Kaveri is a Senior Manager, Data Science at Axtria. She has 10+ years of experience working across the globe with multiple clients across multiple business domains. Having honed her skills at prestigious organizations such as BCG and UHG, she brought her expertise to the cutting-edge environment of Axtria where she currently serves as Senior Manager. Her tenure at these powerhouses has not only broadened her horizons but also equipped her with a unique blend of strategic vision and hands-on experience. At the heart of Kaveri's prowess lies her deep expertise in Machine Learning Engineering and MlOps. As a thought leader, Kaveri has become a driving force behind the seamless integration of machine learning models into the operational fabric of organizations.
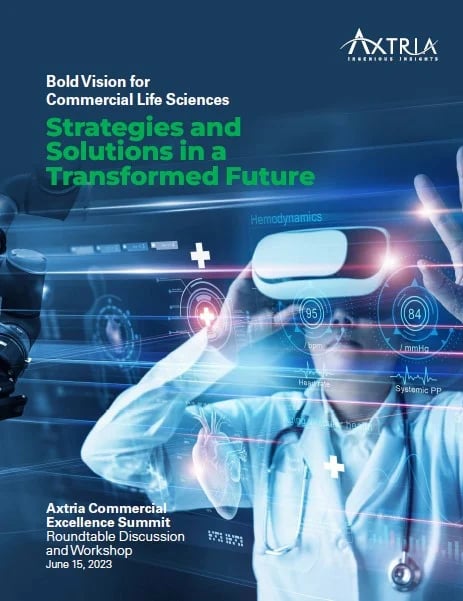
Complete the brief form to download the white paper
Related insights
Stay current on topics you care about
Recommended insights
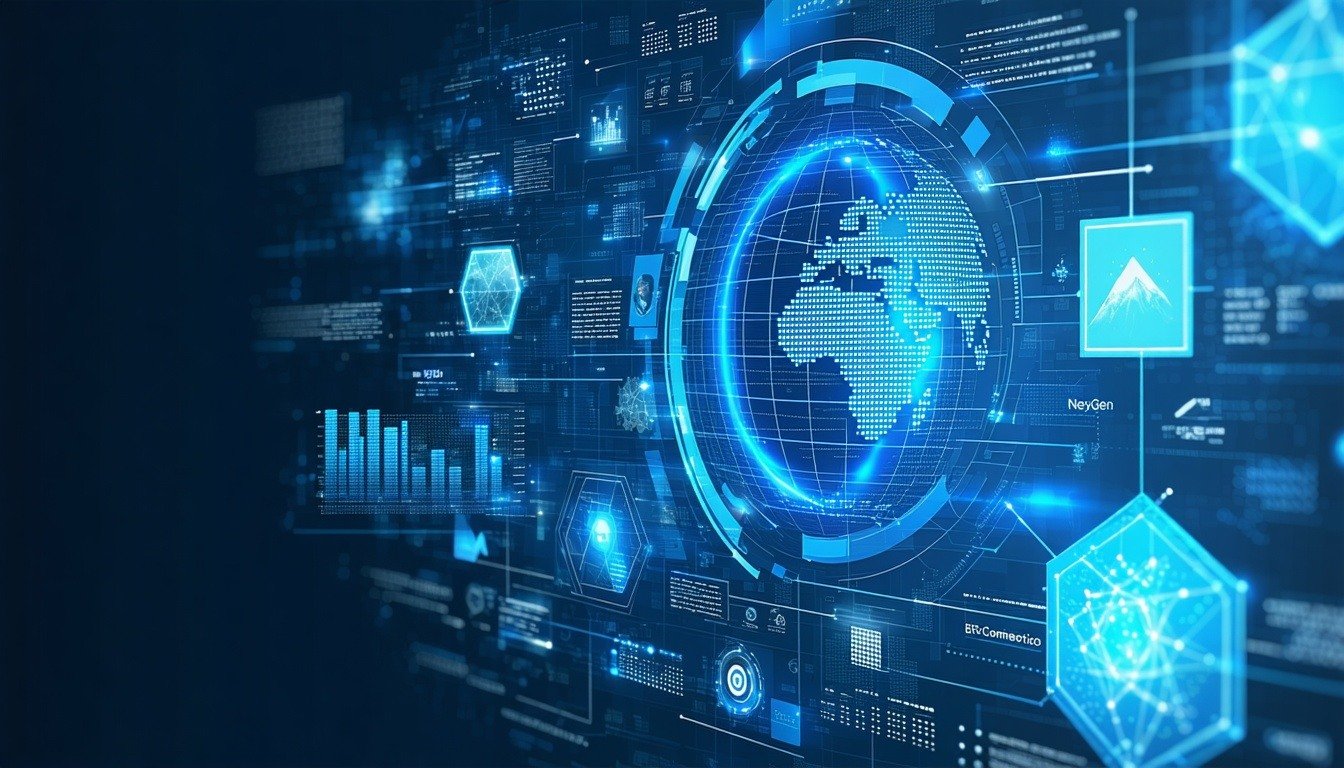
Blog
NexGen Commercial Intelligence: How Generative and Agentic AI are powering Field Force Effectiveness
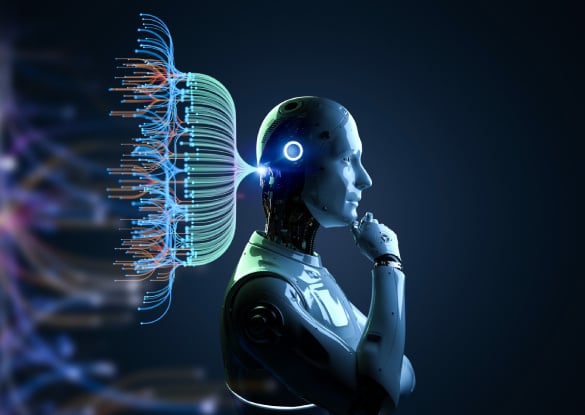
Article