All Insights Article Pharma’s Most Innovative Research Assistant Is Powered With Generative AI
Pharma’s Most Innovative Research Assistant Is Powered With Generative AI
Pharmaceutical research activities are crucial drivers of innovation. Researching for therapy area nuances, industry-wide developments, and medical science breakthroughs serves several objectives. The goal is to discover and develop new medications and treatment strategies. In-depth research into the biological mechanisms of diseases uncovers vulnerabilities that can be exploited for therapeutic intervention. However, the focus of research extends beyond groundbreaking discoveries.
A significant portion of research is dedicated to refining existing medications. This can involve minimizing side effects, enhancing therapeutic efficacy, or developing more convenient delivery methods. Continuous research helps optimize well-established treatment pathways, making them more efficient, tolerable, and beneficial for patients.
This article delves into traditional research methodologies, their inherent challenges, and the revolutionary potential of generative AI (GenAI)-based research assistance tools while illustrating an example of research for the non-small cell lung cancer (NSCLC) therapy area.
Traditional Research Methods in Pharmaceutical Companies
Pharmaceutical research traditionally relies on exhaustive manual processes. Researchers spend countless hours poring over medical journals, clinical trial data, health economics reports, and real-world evidence (RWE) studies. These activities are typically segmented into the following essential tasks:
-
Data collection: Gathering information from many sources is the first critical step. Researchers collect data from academic journals, clinical trial results, internal databases, health records, regulatory documents, and various online databases. This involves extensive searching, accessing, and organizing data that is often spread across multiple platforms.
-
Literature review: Researchers engage in rigorous literature reviews once data is collected. This process involves reading and analyzing many published studies to stay updated on the latest findings, theories, and developments. It requires critically evaluating each piece of literature’s relevance, quality, and implications.
-
Data synthesis: After the literature review, researchers compile and synthesize the data to form a coherent understanding of the current state of research. This synthesis involves comparing and contrasting findings, identifying trends, gaps, and inconsistencies, and integrating diverse data sources to generate comprehensive insights.
-
Reporting: The final step involves summarizing the synthesized data into detailed reports. These reports provide actionable insights and recommendations, supporting further research and development efforts. They are crucial for decision-making, guiding the direction of new studies, informing drug development processes, and ensuring compliance with regulatory standards.
Researching relevant medical information is tedious. Several challenges make the overall process inefficient and impact the comprehensiveness and accuracy of the research results. Some prominent difficulties include:
-
Time-consuming: The manual nature of traditional research methods makes the process extremely time-consuming.
-
Volume of data: The sheer volume of available research papers and data can be overwhelming, making it challenging to keep up with the latest developments.
-
Relevance and accuracy: Identifying the most relevant studies and ensuring the accuracy of the extracted information is prone to human error.
-
Contextual understanding: Traditional search tools, such as those based on keyword searches, often cannot understand the context of queries, leading to the retrieval of irrelevant information.
The Potential of GenAI-Based Research Assistants
GenAI’s potential as a research assistant in pharmaceutical research is noteworthy. By leveraging natural language processing (NLP) and large language models (LLM), GenAI can significantly enhance the efficiency of the research process. Here’s how GenAI can transform the role of research assistants:
-
Enhancing data collection
-
GenAI can automate the manual gathering of information from diverse sources, quickly aggregating relevant data from multiple platforms. This saves time and ensures a more comprehensive data collection effort.
-
GenAI tools can continuously ingest data in real time, ensuring researchers can access the most up-to-date information. This capability is crucial in the fast-paced world of pharmaceutical research, where new findings and data are constantly emerging.
-
-
Improving literature review
-
GenAI can swiftly process and summarize large volumes of text, identifying key insights and trends. This allows researchers to stay current with the latest knowledge without information overload.
-
GenAI’s NLP capabilities enable it to understand and interpret complex scientific language. Researchers can use natural language queries to interact with AI systems, making retrieving specific information from extensive datasets easier. This reduces the time spent on searching and allows for more focused analysis.
-
-
Facilitating data synthesis
-
GenAI excels in integrating and synthesizing data from multiple sources. GenAI can identify patterns, inconsistencies, and gaps in existing research. This holistic view of the data helps form a coherent understanding of the current state of research, which is essential for guiding new studies and supporting drug development processes.
-
Beyond essential data integration, GenAI can perform complex analyses that might be challenging for human researchers. It can apply statistical models, predictive analytics, and other advanced techniques to generate deeper insights from the data.
-
-
Enhancing reporting
-
One of the most significant contributions of GenAI is its ability to generate concise and detailed summaries of complex data. Researchers can rely on GenAI to produce comprehensive reports that provide actionable insights. This speeds up the reporting process and ensures high accuracy and clarity.
-
GenAI-generated reports can include recommendations and predictions based on the analyzed data. This proactive approach helps researchers and decision-makers identify opportunities and challenges early, guiding further research and development.
-
Example: Researching NSCLC With GenAI Q&A Tools
NSCLC is a prevalent and high-mortality form of lung cancer. Staying up to speed on the latest research is crucial for developing effective therapies. GenAI-based question and answer (Q&A) tools can significantly enhance the research process. Once the initial step of curating a comprehensive database of medical journals, clinical trials, and other relevant documents is completed, the GenAI system can be trained on the data to understand and process queries related to NSCLC. For instance:
-
“What are the side effects of the most commonly used chemotherapies for NSCLC?”
-
The tool would list common side effects of chemotherapeutic agents, along with their severity and management strategies.
-
-
“How does immunotherapy compare to traditional chemotherapy in treating NSCLC?”
-
The GenAI tool would compare the effectiveness, side effects, and patient outcomes of immunotherapy versus chemotherapy, supported by clinical trial data and real-world evidence.
-
-
“What lifestyle changes can improve the prognosis of NSCLC patients?”
-
The tool would offer recommendations on lifestyle modifications, such as diet, exercise, and smoking cessation, based on the supporting evidence from research studies.
-
-
“What are the current guidelines for the early detection of NSCLC?”
-
The GenAI tool would outline screening recommendations, including criteria for high-risk populations, types of screening tests, and their efficacy in early detection.
-
-
“How do socioeconomic factors impact NSCLC treatment outcomes?”
-
The GenAI tool would discuss the influence of factors like income, education, and access to healthcare on the diagnosis, treatment, and survival of NSCLC patients, supported by relevant studies and data.
-
These examples illustrate the broad range of specific, actionable information that GenAI can provide to support NSCLC research and treatment.
Ensuring Responsibility and Reliability With GenAI
Guardrails and disclaimers are essential to ensure the reliability of GenAI-based tools. These include citations and references in every response, allowing researchers to verify the sources of information. Regular updates to the database are necessary to maintain the relevance and accuracy of the information by incorporating the latest research. Data can be gathered from websites using web crawlers or scraping techniques, ensuring a comprehensive and current dataset. Handling complex queries requires advanced algorithms to manage multi-faceted questions and provide synthesized answers. Data privacy is crucial, and GenAI tools must guarantee the confidentiality of sensitive patient data. Compliance is also substantial, and AI tools can ensure that industry regulations, such as FDA drug development and research guidelines, are being met.
The integration of GenAI Q&A tools in pharmaceutical research represents a paradigm shift, promising to enhance efficiency, accuracy, and depth of insights. In the case of NSCLC, such tools can provide researchers with rapid access to the latest developments and a more comprehensive understanding of the disease and its treatments. As the life sciences industry evolves, adopting these technologies will drive innovation and improve patient outcomes.
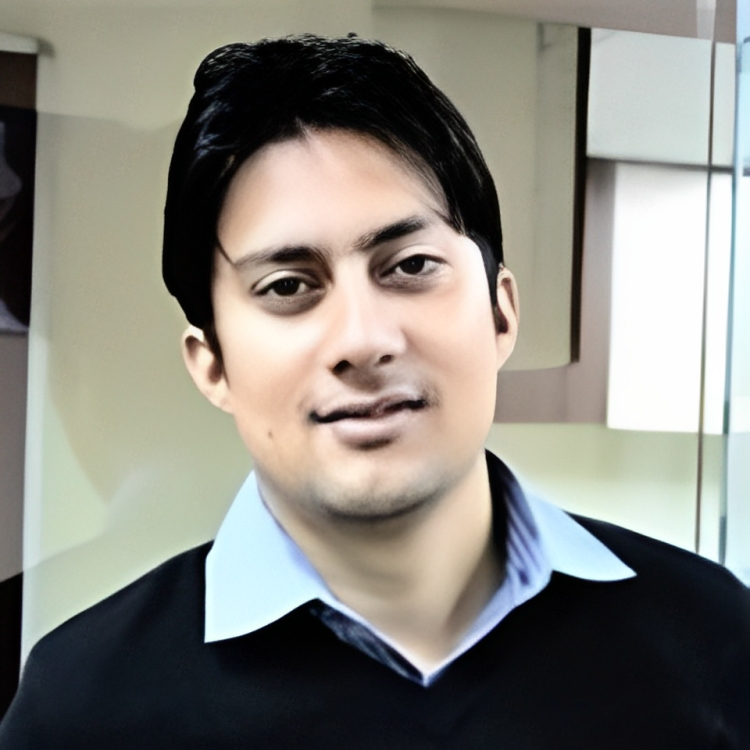
Rajeev Bhardwaj
Rajeev is a Solutions Architect with the Axtria Technology Center of Excellence team. His expertise spans generative AI, large language models, Big Data engineering, business information management, cloud computing, and advanced analytics.
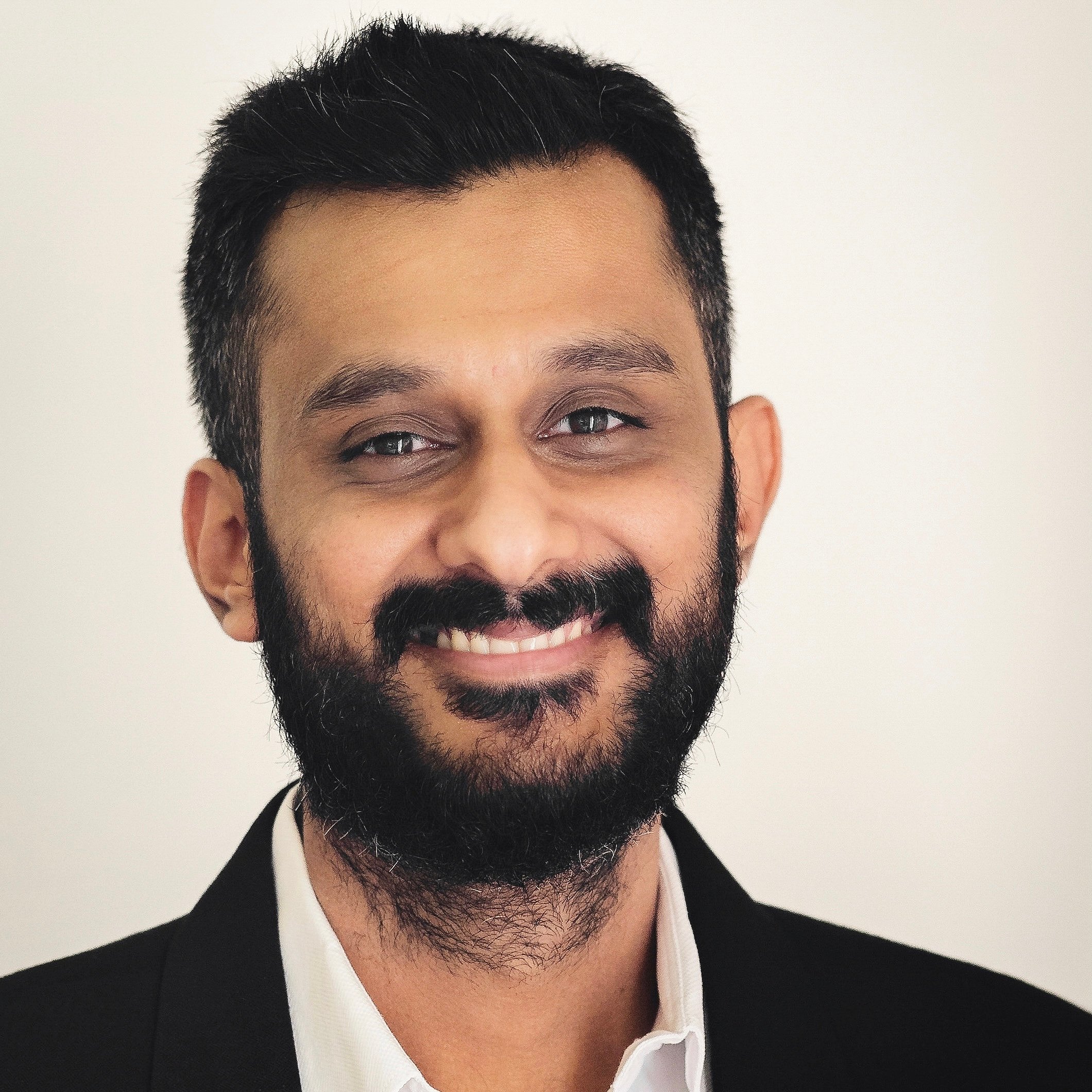
Suraj Gupta
Suraj Gupta is a Senior Manager, Marketing at Axtria. He has over 9 years of experience in the analytics and consulting industry, with more than 6 years with life sciences. At Axtria, Suraj has contributed to sales and marketing functions, including business and client development, knowledge management, digital marketing, business intelligence, and content writing. Suraj has an MBA degree in Marketing and a graduate degree in Economics.
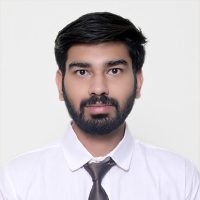
Nikhil Ojha
Nikhil is a Senior Developer with the Axtria Technology Center of Excellence team. His expertise spans Machine Learning, ML Ops, generative AI, Big Data engineering, cloud computing, and advanced analytics.
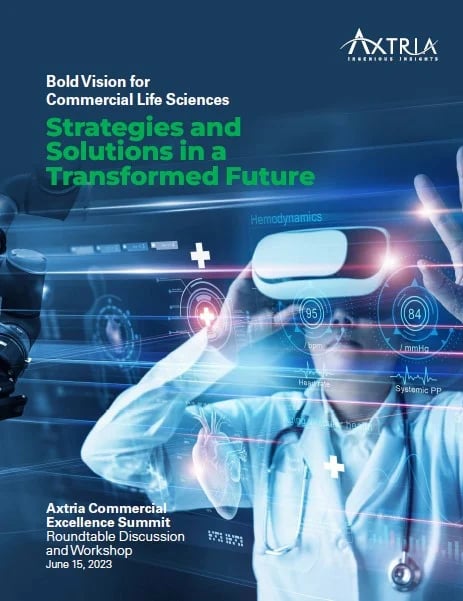
Complete the brief form to download the white paper
Related insights
Stay current on topics you care about
Recommended insights
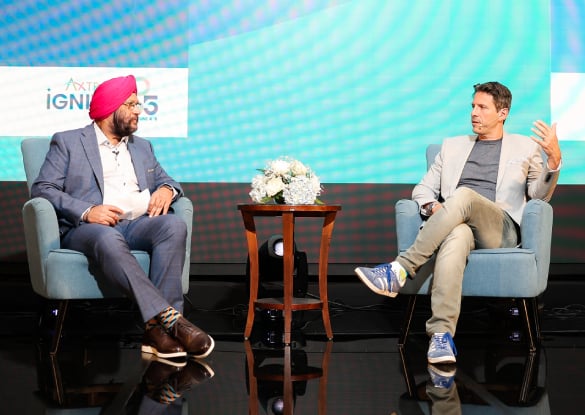
Article
Axtria Ignite 2025: Inspiring Innovations, Building Bonds, And Advancing Life Sciences With AI
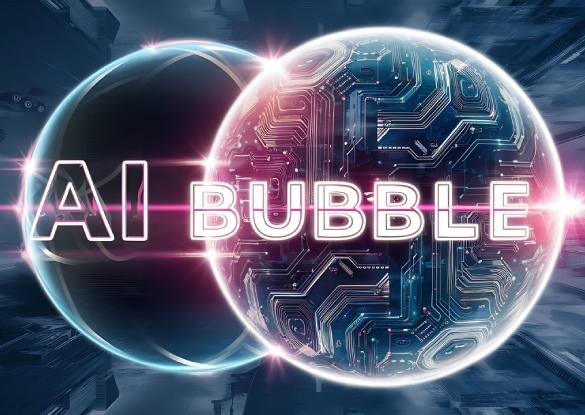
Article
Beyond the Large Language Model Hype: Creating Sustainable Patient Value in an Era of Continuous Generative AI Evolution
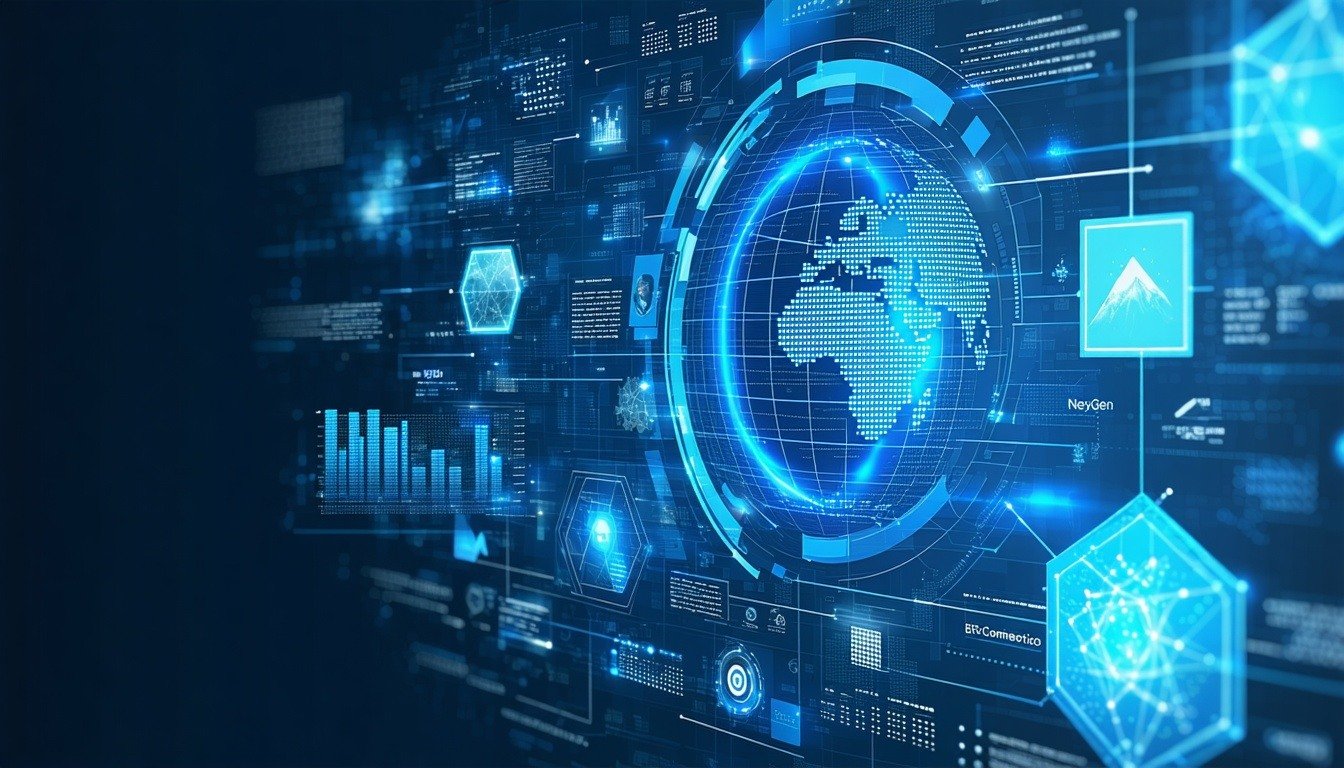
Blog