Problem
Rare diseases, also known as orphan diseases, affect a small percentage of the patient population (<200,000 patients in the US but usually much lower) with much higher drug therapy treatment costs than average [1]. Rare diseases present numerous challenges from a marketing perspective; however, none are more challenging than deciding whom to target for details, and when (or if) to consider stop detailing to targets who have not used a client product after two, three, or even five years of sustained detailing. While sales forces for rare disease products are much smaller than those for widespread disease products, they are frequently in the range of 30-150. The uncomfortable fact for rare disease marketers is that their largest promotional spend will almost certainly be the cost of details to healthcare professionals (HCPs) who have never written a script for their product, regardless of the phase of the lifecycle, market competition, or anything else. It is not uncommon to have 75% of targets for rare disease products not writing scripts for the product three, four, five years after launch.
Many pharmaceutical companies have traditionally taken somewhat of a "shotgun" approach to face-to-face promotion resourcing for rare diseases. They cast a wide net to target as many HCPs in relevant specialties that have any chance of seeing a relevant patient. From a purely financial perspective, this strategy generally makes sense given the imbalance between the gross-to-net (GTN) lifetime value (LTV) of new patient starts and the costs of planned details. The LTV of a new patient start can easily be 10 to 100 times the projected cost of three - five years of detailing. However, a disproportionate number of HCP targets for personal promotion in rare disease markets are likely to have never written a script a few years into brand launch. When and how to decide when to give up on some of those non-writers, and reduce the enormous associated prospecting costs, are key questions this blog will address.
Many pharma companies seem to be fine with these planned “prospecting” costs given the large disparity between the LTV of a new patient start and the costs of planned details, given the sneaking suspicion/hope that even the most incalcitrant targets may one day turn the corner and write a script. However, many others want, and sometimes need, to get more precision on when to stop, or lower, the detailing investment for non-writers.
Solution Framework
One of the robust approaches for achieving targeting precision concerning non-writers is to calculate the expected value (EV) of continued detail investment for each target. The EV calculation should be based on an ensemble of machine learning (ML) HCP adoption model and brand/promotion financials. Mathematically, prospecting for a non-writing target in any market should continue if the value for equation (1) below is greater than 1.0. That is, for all non-writers, consider stopping or at least reducing future detail investment when the EV for future planned investments in details is less than 1.0. These EVs should be calculated quarterly for all non-writers.
P(Adopt)i,t1* GTN-LTVnew start / Planned Detailsi,t1* Cost Details, Where:
P(Adopt) i,t1 = probability non-writeri, writing in future
GTN LTV New Start = GTN LTV of a new patient starts
Detaillsi,t1 = planned details to target in the future
Cost = detail cost
The key ingredient to solve for the equation (1) is P(Adopt) i,t1 - the probability of adopting a non-writing HCP target. Robust adoption models require a wide array of features from diverse data assets spanning from patients, payors, physicians, institutional controls, macro-economic/social, and other factors [2], and are almost always generated using the ensemble of two - three alternative modeling techniques such as Random Forests, Support Vector Machines (SVM) or Convolutional Neural Network (CNNs).
Figure 1: AXTRIA’S HCP ADOPTION MODELING FRAMEWORK
Source: Axtria Inc.
The Answer
Clients should ”consider giving up” detail investments for non-writers when the expected value (EV) for future planned investments in details is less than zero. We have found that up to 25% of non-writing targets have EVs of planned details in the next two quarters below zero. While it is common for many rare disease products to have few or no, non-writing targets with EVs < 1.0, we almost always find clear differentiation among non-writing targets on EV that can be used to more clearly prioritize non-writers for future detail investment. Note that this is a first pass approximation that must be tempered considering other information about the HCP and/or data imperfections. For example, a HCP with EV < 1.0 who refers patients for an injectable product but does not show as a writer in claims data should almost certainly remain a target for details.
The initial question is not permanently solved with ML adoption-model based EV calculations with the judicious application of common-sense business rules. Once those qualitative factors are taken into account, the key to driving enhanced value from this targeting algorithm is to use reinforcement learning/adaptive updating to ensure that targeting decisions evolve as the underlying conditions for the behavior evolve over time.
Application & Business Benefit
The most obvious benefit of using a robust EV approach to drive decisions on when to give up the ghost on non-writers is the potential resources freed up for more profitable investments. Details diverted from low EV non-writers could be directed to potentially more profitable non-writers, or to writers identified as responsive to incremental details. The frequent substantial details costs to low EV non-writers could, in principle, be shifted to other underfunded non-personal promotion (NPP) activities.
However, before ”giving up” on any targets, primary research should be conducted to understand the underlying needs, attitudes, and beliefs (NAB) for all targets to identify tailored messaging for high potential, low EV non-writers, and executing against those insights. How to do this well for the rare disease markets will be the second blog in this series.
If you are interested in learning how targeting precision can benefit rare disease drugs, please contact us (directly below). Axtria would be delighted to help and ensure that your commercial analytics team is prepared for the challenges involved with rare disease targeting while ensuring that HCPs are engaged in a more productive fashion.
For additional information on rare disease targeting, please explore the Axtria Research Hub and Axtria Blogs.
References
- Rare Diseases - Challenges Associated With Orphan Drug Commercialization: https://insights.axtria.com/infographics/rare-diseases-challenges-associated-with-orphan-drug-commercialization
- Sravan Kumar Bhamidipati, Vijay Chickarmane, PhD. PMSA Machine Learning Applications in Commercial Life Sciences by Axtria. https://pmsa.org/past-symposia-presentations/2019-symposium/1631-2019Symposium-06-Axtria/file
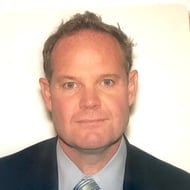
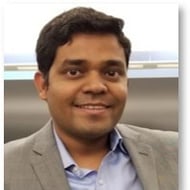