All Insights Article The Growing Influence Of AI In MedTech
The Growing Influence Of AI In MedTech
Intro
Over the past few years, artificial intelligence (AI) has seen massive technological advancements and increasingly widespread industry adoption, leading it to become one of the most talked-about topics in the life sciences. Meanwhile, the MedTech field is beset by a number of unique challenges that make the old way of doing business seem increasingly obsolete. While it is essential that all life sciences professionals learn how to use AI effectively, MedTech manufacturers, in particular, should understand what AI is, how it can be applied to the hurdles they face, and the pros and cons of its usage.
The Basics of AI
Artificial intelligence is a broad and nebulous term encompassing several disciplines of computer science dedicated to teaching computer programs how to process and generate information the way a human brain does. In an industry context, it can be helpful to think of it as an advanced data analysis tool that emulates what human analysts or technicians do, but at a much quicker pace and, in many cases, with greater accuracy.
Much like a human brain, AI “learns” by taking in information and picking up on common patterns while being trained. It can later look for those patterns when it receives similar information. Data scientists feed data to an AI in incredibly high volumes during the training process. For example, a 2017 study of a diagnostic AI model used 129,450 images of cancerous skin as its training data.1 A series of connected nodes called a neural network (so named because it mirrors the structure of neurons in the brain) gradually adjusts its paths between relevant nodes, enabling the AI to identify and categorize new data more effectively.2
There are two basic types of AI training models. In supervised learning, an AI’s training data includes descriptive labels because the programmer wants the AI to distinguish between existing categories. Direct feedback from the labels helps the AI check itself. In unsupervised learning, the programmer wants the AI to identify hidden patterns in the data. Labels are not included so they won’t interfere with the discovery process. AI models designed to learn information and apply those learnings are typically classified as machine learning models. Those with especially intricate neural networks may be classified as deep learning.3 AI can be trained on many different types of data, from images to written language, as long as the data can be digitally processed and patterns can be observed. While the applications and types of machine learning are myriad, its purpose is consistent across the board – take in high volumes of data, learn how the data work, and use that knowledge to process, make predictions for, and generate new data.
The Role of AI in MedTech
While machine learning is far from new, it has entered its golden age in recent years, becoming much more robust and, consequently, more widely used. MedTech is no different; while the FDA’s archives indicate that the first medical device incorporating machine learning-like tools was approved in 1995, more AI-powered devices have been approved since 2020 than in the entire two-and-a-half decades before.4 In response to the increase in AI-powered medical technology and the inherent difficulty of conducting a one-time evaluation of software that learns and evolves gradually, the FDA began requiring that MedTech companies submit a Predetermined Change Control Plan for AI-powered devices in 2019 that details how the algorithm will evolve over time.5 Since then, hundreds of AI-powered devices have successfully undergone this combination of the FDA’s standard medical device approval processes (typically 501(k)) and the Predetermined Change Control Plan review.
AI is uniquely well-suited for diagnostic analysis of medical images, as an AI model can easily be trained on extant images of a particular condition and consequently identify visual signs of that condition in patients. The aforementioned 2017 study developed an AI model that could diagnose specific skin cancer conditions as accurately as board-certified dermatologists; AI diagnostic technology has only become more widespread and effective since then.6 Seventy-five percent of AI-powered medical devices currently on the market are classified by the FDA as radiology devices and use similar functionality to aid in the diagnostic process.7 Their role in the process varies; some will diagnose conditions wholesale, while others may simply highlight abnormalities. Whatever their abilities, various studies have indicated that incorporating AI models into human-led diagnostic processes yields better results than humans or diagnostic AI alone.8
Another popular application of AI is conversational chatbots. You may be familiar with ChatGPT or similar products like Google’s Bard AI or Axtria’s secure DANI Field Intelligence chatbot – these are examples of large language models that use written language as their training data and effectively learn to speak in a human-like cadence about various subjects. Language models with medical care-oriented training can be incorporated into digital health software as a medical device (SaMD) to provide patients with personalized care, encourage adherence, and help patients schedule appointments in a convenient and time-efficient manner. Additionally, AI can expedite remote monitoring by learning how healthy individuals typically behave and consequentially identifying out-of-the-ordinary behavior from video feeds, making treatment faster and more effective.
AI can also be beneficial in the sales process, improving efficiency in both planning and communication. Managing sales can be uniquely arduous for MedTech companies, as they not only have to deal with high volumes of customer data from a broad variety of sources and formats, some of which can be difficult to parse, but they must also adjust quickly to abrupt market shifts caused by increasingly frequent mergers and acquisitions activity and reorganization. Meanwhile, traditional sales planning methods incorporate statistical methods that require heavily structured data and tend to assume that the relationships found in the data will fit a specific model, making it difficult to identify atypical but significant customer trends and reach out to these customers accordingly. Alternatively, AI-powered data analysis can track relationships simply without forcing them into a particular model or structure. This results in greater accuracy during analysis-heavy processes like segmentation, demand forecasting, and lead generation. In fact, a 2001 study comparing conventional mail targeting done by humans and targeting done by a simple neural network found that the latter had a 70% response rate compared to the former’s 30%. The fact that such remarkable results occurred even with the primitive forms of AI available in 2001 is a testament to how well-equipped AI is to handle the sales planning process.9
At the same time, generative AI models can be incredibly helpful to salespeople during actual communication with clients, as AI can process unstructured, hard-to-parse information such as transcripts of sales calls, emails, or clients’ prescribing data. Such models can provide effective, personalized client communication recommendations, resulting in improved outcomes. AI can also make administrative tasks easier, as it not only evaluates sales reports at face value but also uses them to recommend ways to improve salesperson performance. AI trained on company records should thus be integrated into all aspects of the sales process to minimize time spent on rote work and increase positive results and adaptability for organizations confronting an ever-changing market.
Important Considerations
As with any technology, AI has its pros and cons. One of the most common criticisms of AI is its tendency to reflect biases acquired from the training data. Medical data often underrepresent certain demographics, and even when representation is adequate, makers of AI-powered medical devices may fail to thoroughly label their training data, making their devices ill-equipped to diagnose conditions that manifest differently in certain patients.10 This produces inaccurate results and may alienate consumers from AI-powered devices due to the perception that they “only work for certain people.” To counter this impression, medical device manufacturers should set comprehensive standards for well-labeled and diverse training data and be transparent with the public about these standards. This will improve perceptions of AI-based products and ensure that those who use AI-based medical devices can understand how they work and thus use them more effectively.
Just as mismanaged AI can enforce imbalances in the medical device industry, cautiously managed AI may be able to minimize them. Early adoption of AI in the Chinese MedTech industry was motivated by healthcare inequity across urban and rural areas, as rural clinics often lacked funding, staffing, and access to technology compared to their urban counterparts. Because they now entrust some of their work to AI, rural clinics can offer more services and communicate more effectively without placing strain on their limited resources.11 This results in more efficient, less costly, and more accessible healthcare – indeed, the World Health Organization notes that “AI-based systems could reduce the number of hospital admissions and overall healthcare costs without reducing the quality of patient care.”12
It is inarguable that AI has already massively impacted the MedTech industry, and its impact will only grow in the coming years. It is estimated that 50% of organizations in the life sciences space will have adopted AI technology in some form by 2025, and those that do adopt AI stand to increase profits significantly; a projection from McKinsey estimates that the use of AI could generate upwards of $100 billion for the healthcare industry as a whole.13 Thus, it is essential that MedTech companies find ways to integrate the power of AI into their business or risk being behind the curve and missing out on profits. Of course, the novelty of this technology, as well as the technical expertise necessary to use it properly, makes this a complicated task. A report by Harvard Business Review recommends that companies hoping to integrate AI into their workforce outsource the technical work while designating certain internal employees as “boundary spanners” to communicate between the sales world and the AI world, as developing AI capabilities in-house can be an expensive, complex, and ultimately unnecessary ordeal.14 Many companies, Axtria included, have developed advanced AI-based analysis methods and software to make the transition to an AI-powered workforce easier for our clients. Harnessing the power of AI may be a demanding task, but the remarkable growth of the field in recent years has made an AI-powered future for MedTech a foreseeable reality.
REFERENCES
- Basu K, Sinha R, Ong A, Basu T. Artificial intelligence: how is it changing medical sciences and its future? Indian Journal of Dermatology. 2020;65(5):365. doi:10.4103/ijd.ijd_421_20
- Scharre P, Horowitz MC. Artificial intelligence: what every policymaker needs to know. Washington, DC: Center for a New American Security; 2018.
- He J, Baxter SL, Xu J, Xu J, Zhou X, Zhang K. The practical implementation of artificial intelligence technologies in medicine. Nature Medicine. 2019 Jan 7;25(1):30–6. doi:10.1038/s41591-018-0307-0
- Artificial intelligence and machine learning (AI/ML)-enabled medical devices. Washington, D.C.: U.S. Food & Drug Administration; 2022.
- Center for Devices and Radiological Health. Artificial intelligence/machine learning (AI/ML)-based software as a medical device (SaMD) action plan. Washington, DC: U.S. Food & Drug Administration; 2021 p. 1–7.
- Syam N, Sharma A. Waiting for a sales renaissance in the fourth industrial revolution: machine learning and artificial intelligence in sales research and practice. Industrial Marketing Management. 2018 Jan 17;69:135–46. doi:10.1016/j.indmarman.2017.12.019
- Malpani R, de la Fuente-Núñez V. Ageism in artificial intelligence for health. WHO Policy Brief Geneva: World Health Organization; 2022 p. 2–13.
- McGrail S. AI in the pharma industry: current uses, best cases, digital future. Pharma News Intelligence. April 30, 2021. [Accessed July 25, 2023]. https://pharmanewsintel.com/news/ai-in-the-pharma-industry-current-uses-best-cases-digital-future
- Sinha P, Shastri A, Lorimer SE. How generative AI will change sales. Harvard Business Review. March 31, 2023. [Accessed July 25, 2023]. https://hbr.org/2023/03/how-generative-ai-will-change-sales
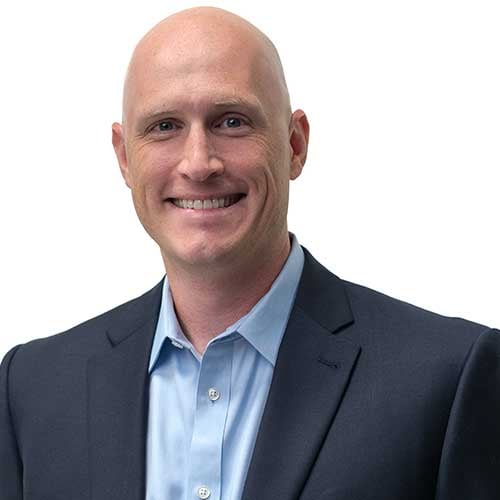
Brian C. Rogers
Brian C. Rogers is a Director of Commercial Excellence at Axtria. Brian previously worked in the med-tech market, including tenure at a major medical device manufacturer in the finance/accounting function and leadership roles in reporting, analytics, and sales operations. He also spent over 10 years consulting in the healthcare provider space and has several years of experience leading the business intelligence department at a top insurance company. Brian holds a BS in Management Science and Information Systems from The Pennsylvania State University’s Frank P. and Mary Jean Smeal College of Business Administration and an MS in Information Systems, and an MBA from DeSales University.
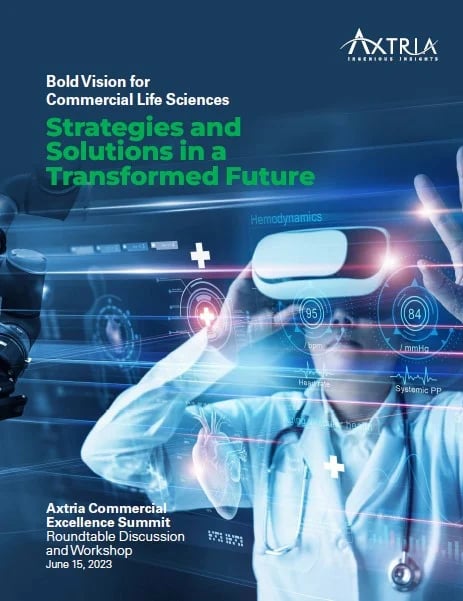
Complete the brief form to download the white paper
Related insights
Stay current on topics you care about
Recommended insights
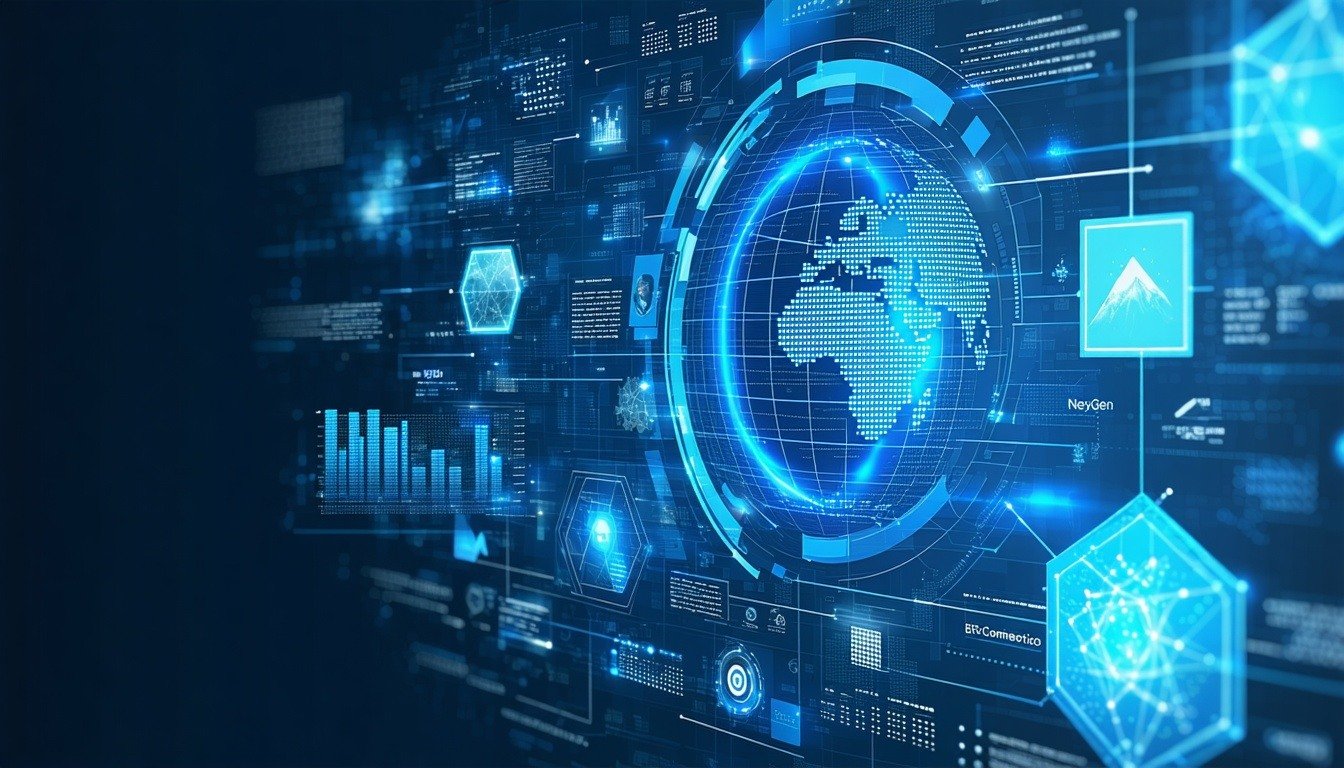
Blog
NexGen Commercial Intelligence: How Generative and Agentic AI are powering Field Force Effectiveness
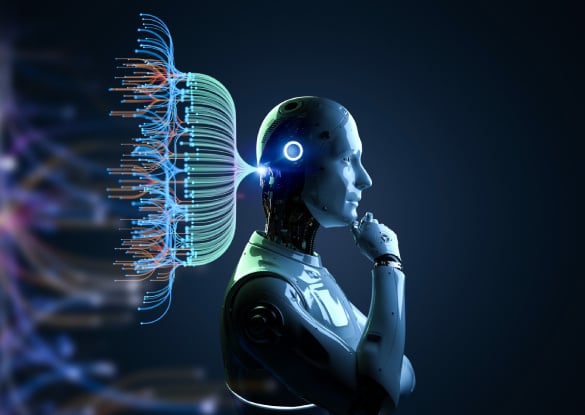
Article