The world of health and medicine has broken countless barriers over the last few scores. Life sciences companies have continued to invest significantly in their research of globally corroding diseases, drug molecules discovery and development, and closely monitored clinical trials, to create industry-approved blockbuster medicines. These drugs, gaining precision with time, are curing more diseases and giving patients a chance at better lives. The pharma journey, however, gets complicated with more in-depth clinical and genetic research, as companies continue to find themselves facing more significant ‘unknowns’ than ever before.
What are Rare Diseases?Rare diseases came to be known as orphan diseases as, traditionally, few life sciences companies were interested in adopting them to develop treatments. The Orphan Drug Act of 1983 was launched to incentivize pharma companies to shift their focus towards such diseases. |
Rare diseases, also known as orphan diseases, are those that affect a small percentage of the patient population1. In the U.S., rare diseases are defined as those affecting 200,000 or less or about 1 in 1,650 people. In the European Union, a disease is considered rare if it affects less than 1 in 2,000 people2. The origin of such diseases is mostly unknown, but most (about 80 percent3) are known to be associated with genetic changes and complications. These diseases carry a wide array of disorders and symptoms that not only differ from diseases but also people carrying the same disease4. For more details, refer to Axtria’s infographic on rare diseases.
Over the past few years, life sciences companies have made commendable advancements in the realm of rare diseases, but the dominance of the associated challenges still exists. These challenges can range from ‘rare disease awareness’ to the ‘holistic treatment of patients.’ More so, it can take up to 7.6 years for a patient to be accurately diagnosed with a rare disease5. This period lag is grossly unacceptable and needs serious intervention. If patients are not correctly diagnosed at the appropriate stage of their disease cycle, several treatment opportunities are eliminated, let alone the limited treatment options available. Pharma and biotech companies have recognized this fact and are investing heavily in shortening the diagnosis cycle of rare diseases. By leveraging cutting-edge technology to better understand rare diseases, some of the top pharma companies are centrally focusing on predictive patient identification, accurate disease diagnosis, and ahead-of-the-curve treatment options.
The advent of artificial intelligence/machine learning (AI/ML) and its associated capabilities create several opportunities for intelligent intervention, which, if leveraged correctly, can significantly improve the rare disease treatment journey. This blog examines three such areas, and how AI/ML can play a pivotal role to expedite the accurate patient identification and diagnosis process.
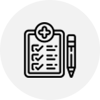
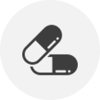
Markers
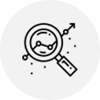
Patient Data
What is Protected Health Information (PHI)?Protected Health Information (PHI) is any medical information that is used to identify individuals based on their health records, for healthcare services such as diagnosis or treatment. This information is HIPAA-compliant.6 |
Patient-level data is available in abundance today, arriving in both structured and unstructured formats. Companies are mining patient-level data from sources such as devices (wearables and smartphones), digital platforms (social media and search engines), and medical records (Electronic Health/Medical Records - EHRs/ EMRs and Real-World Evidence - RWE). Patient information from all these data sources is collectively used to create Protected Health Information (PHI) records, by intelligently integrating the structured and unstructured data into a unified single-source-of-truth.
Pre-defined business rules, along with AI/ML techniques such as Natural Language Processing (NLP) and Text Mining, help arrange the PHI master data into multiple logical disease indicators. Consisting of claims, diagnostic, and prescription information, each of these indicators aid in a better understanding of disease complexities. By narrowing down the scope to specific rare disease cases, these flags have the potential to create an encyclopedia of identifiable rare disease indicators based on real-life rare disease scenarios. Also, by ensuring a continuous feedback loop mechanism, ML algorithms will help make these indicators more accurate over time.
Disease Pattern Makers
Patient indicators can roll up to create disease-specific personas. These personas are based on demographics, symptoms, behaviors, and medical histories of sets of homogenous rare disease patients. Powered by AI/ML-based markers across the disease lifecycle, pre-defined patient personas (or genomes) serve as go-to guides for identifying rare disease indications. Further, advanced statistical techniques will help verify each persona’s correlation with the associated disease.
Additionally, companies can also tie-up with industry-leading physicians to super-impose these algorithms with personal expertise in dealing with such patient populations. As a result, accurate disease pattern markers can be derived, which are backed by deep patient data analysis, AI/ML-based algorithms, and expert supervision. When used along with predictive triggers over ongoing patient monitoring, these flags add immense value to the diagnosis of rare disease patients.
Predictive triggers
The disease identification mechanisms always work at two levels – the patient and physician. When equipped with the right knowledge, intelligent technology, and due diligence, such mechanisms work wonders in saving lives. Pre-defined disease markers will form an integral part of the diagnosis chain in the future. Embedded across multiple patient tracking devices such as wearables and smartphones, and integrated with physician reports and dashboards, disease markers will help raise flags at the onset of the slightest disease symptoms. AI/ML-driven triggers can closely monitor patients around the clock and predict rare disease indicators very early in the diagnosis process, shortening the overall diagnosis timeline. When alerted, patients and physicians will be able to work together to remove all possibilities of disease morbidity and take the necessary steps to improve patient outcomes.
Conclusion
Studying the right kind of data, deriving actionable insights, and integrating them into a sustainable people-driven operational strategy is the only way for life sciences companies to demystify rare diseases. A better understanding of patient journeys will continue to shorten (and strengthen) disease diagnoses. Life sciences companies need to work closely with healthcare stakeholders to include patients in their diagnostic cycles. With appropriate data and assisted technology, patients and physicians can work together to create better treatment options, and improved patient lives.
The inception of AI/ML and its limitless sea of computing possibilities has encouraged life sciences companies to embrace the fourth industrial revolution. With this, the trifecta of data, technology, and people, promises to break bigger healthcare barriers, as it has in the past.
REFERENCES
- Eurordis, The Voice of Rare Disease Patients in Europe: https://www.eurordis.org/content/what-rare-disease
- NIH, FAQs About Rare Diseases: https://rarediseases.info.nih.gov/diseases/pages/31/faqs-about-rare-diseases
- America’s Biopharmaceutical Companies, Rare Disease by the Numbers: https://innovation.org/about-us/commitment/research-discovery/rare-disease-numbers
- Eurordis, WHAT IS A RARE DISEASE?: https://www.eurordis.org/sites/default/files/publications/Fact_Sheet_RD.pdf
- BMC, Orphanet Journal of Rare Diseases: https://ojrd.biomedcentral.com/articles/10.1186/s13023-019-1075-8
- HIPAA Journal, What is Protected Health Information?: https://www.hipaajournal.com/what-is-protected-health-information/