All Insights Article Beyond Boundaries: Reshaping Pharma’s Commercial Horizon with MLOps
Beyond Boundaries: Reshaping Pharma’s Commercial Horizon with MLOps
In the ever-evolving landscape of pharmaceuticals, achieving commercial excellence is no longer just an ambition but a dedicated endeavor. The synergy of data, artificial intelligence (AI), and machine learning operations (MLOps) drives the industry toward an age of unparalleled innovation. In this article, we look at the intersection of technological and business developments within MLOps, poised to reshape the pharmaceutical domain and raise the bar for commercial excellence in this vital field.
MLOps is an ML engineering culture and practice that aims at unifying ML system development (Dev) and ML system operation (Ops).1
At the core of this transformation lies the fusion of eight innovative, strategic insights:
- Revolutionizing the pharmaceutical pricing landscape with real-time dynamic pricing: Thanks to the power of MLOps, real-time dynamic pricing is revolutionizing how pharmaceutical companies approach their pricing strategies. Real-time data analysis and AI models have allowed them to adjust drug pricing swiftly—a profound shift in the industry. This trend will persist as enterprises increasingly rely on AI to juggle variables like market dynamics, competitive forces, and regulatory modifications, leading to establishing more competitive pricing models and enhanced profitability, a cornerstone of achieving commercial excellence.
- Pioneering personalized customer engagement Pharmaceutical enterprises are embracing AI-driven approaches to customer engagement; this is the cutting edge of technological and strategic progress. Advanced AI algorithms meticulously scrutinize patient and physician data to craft highly customized communications and interactions. We expect even more personalization as AI systems become more adept at anticipating customer preferences and requirements. Personalized engagement isn’t merely a technical feat; it is a strategic edge that amplifies commercial excellence and the customer experience through tailor-made pharmaceutical solutions.
- Forging a path to business excellence through data-driven decisions: The lifeblood of MLOps is data – and pharmaceutical firms continue to find new ways to use data from diverse sources. AI-driven predictive analytics is becoming instrumental in drug development, resource allocation, and market tactics. This shift goes beyond technical prowess; it is a strategic evolution that makes data-driven decisions the linchpin for achieving commercial excellence. For instance, Janssen recently implemented an automated MLOps process that improved the accuracy of its patient experience model predictions by 21% and increased the speed of feature engineering by approximately 700%, reducing costs while increasing efficiency.2
- Elevating business integrity through regulatory compliance assurance: Pharmaceutical regulations and standards are constantly changing, and compliance is critical. MLOps is a crucial tool that automates compliance monitoring by using AI models that oversee marketing and sales activities. The boost in transparency and auditing capabilities offers a more comprehensive view of regulatory adherence, protects the integrity of business operations, and upholds the highest benchmarks of pharmaceutical compliance, an indispensable foundation for achieving commercial excellence.
- Enhancing business efficiency through supply chain optimization: AI and MLOps are at the forefront of the pharmaceutical supply chain, introducing novel optimization methods. For instance, Pfizer has used MLOps for supply chain optimization. AI/ML capabilities reduced the cycle time of a critical step in the supply chain by 67%, enabling the production of 20,000 extra doses per batch. With AI’s capabilities, Pfizer can manufacture medicines more quickly and benefit more patients worldwide.3
Demand forecasting models are evolving into highly intricate systems encompassing patient demand, drug shelf life, and logistical intricacies. As we move into the future, pharmaceutical supply chains will operate with unparalleled efficiency, minimizing waste and ensuring critical medications are available to those most in need. The quest for efficiency in the supply chain is both a technical marvel and a paramount business necessity, guaranteeing the timely delivery of essential treatments and contributing to commercial excellence. - Augmenting business support with AI-enhanced customer care: Customer support is a vital component of the pharmaceutical industry. The rise of AI-driven chatbots and virtual assistants has been remarkable, primarily due to their capacity to deliver round-the-clock assistance, address routine questions, guide patients on medication usage, and help healthcare professionals identify treatment options. With continuing advancements in AI technology, these tools are poised to foster enduring customer relationships that ensure customer satisfaction and build trust.
- Unlocking the potential of real-world evidence: The increasing adoption of real-world evidence is a promising trend. MLOps empowers the pharmaceutical sector to harness real-world data, extracting invaluable insights on drug efficacy, safety profiles, and patient results. This technological advancement sharpens commercial strategies and reinforces the pharmaceutical product’s value, significantly contributing to achieving excellence in the field. MLOps exemplifies the synergy between technical insights and business value and drives the industry toward new levels of success.
- The fusion of technical and business capabilities through cross-functional collaboration: The success of MLOps is rooted in the collaboration that happens across departmental boundaries. In the future, there will be an even deeper collaboration between data scientists, IT specialists, marketing and sales professionals, and compliance departments. This collaborative environment guarantees that AI-driven ventures align with commercial objectives while delivering on regulatory and security promises. Cross-functional collaboration isn’t solely a technical prerequisite; it is the connective fabric weaving together the realms of technological and business brilliance.
AutoML and Tools for Further Advancement
In pharmaceutical commercial excellence, automation and scalability are critical to success. Automated ML provides methods and processes that make ML available for non-ML experts, improve ML efficiency, and accelerate research on ML.4 AutoML is a proven automation driver, democratizing the development of models and rendering it more accessible and efficient. Automation makes it possible for pharmaceutical companies to refine their product offerings and marketing strategies more quickly, ensuring they remain in lockstep with the ever-shifting dynamics of the market.
Simultaneously, MLOps orchestration using versatile tools such as Mlflow, Kubeflow, and Apache Airflow streamlines operations, increasing competitiveness. Moreover, the scalability of MLOps, facilitated by cutting-edge technologies like Kubernetes, Docker, and PyTorch, empowers pharmaceutical companies to expand their MLOps endeavors. That helps them meet rising demand and ultimately elevates market performance.
Promoting Accessibility and Inclusivity: Expanding the Horizons of MLOps in Pharmaceuticals
MLOps’ role in democratization is crucial – it extends the pharmaceutical industry’s reach and influence. The advent of low-code and no-code MLOps solutions simplifies intricate processes, enabling a wider range of professionals to develop models and make decisions. This inclusive approach ensures that diverse perspectives are factored into the pursuit of commercial excellence.
The deployment of robust tools and platforms facilitates the end-to-end management of a complete MLOps lifecycle, from data collection to model deployment. This approach creates a comprehensive framework for achieving commercial excellence, underscoring the importance of accessibility and inclusivity in driving pharmaceutical innovation.
Development Operations (DevOps) and Continuous Integration/Continuous Deployment (CI/CD) Pipelines: A Catalyst for Commercial Agility
The seamless convergence of MLOps with DevOps and CI/CD pipelines is a pivotal cornerstone in streamlining commercial processes. This collaboration guarantees the swift deployment of AI models and preserves the agility necessary to meet the dynamic demands of the market.
Moreover, the future will see pharmaceutical companies adopting hybrid and multi-cloud MLOps solutions. These adaptive and redundant platforms empower companies to harness multiple cloud providers’ unique capabilities while upholding data security and regulatory compliance. This forward-thinking approach fortifies their position in a rapidly evolving industry.
Catalyzing Commercial Transformation: Edge Computing in Pharmaceuticals
The pharmaceutical sector is on the brink of a revolution in commercial strategies, all thanks to the game-changing impact of edge computing. Edge computing is a distributed computing framework that brings enterprise applications closer to data sources such as Internet of Things devices or local edge servers.5 This technology enables data processing as close to the source as possible, which is incredibly transformative in remote or resource-constrained environments. It can potentially elevate commercial excellence standards by empowering pharmaceutical enterprises to make informed decisions on the go. This enhances the prompt delivery of essential medications and optimizes supply chain management, making edge computing a pivotal force in reshaping the industry’s future.
The Nexus of Commercial Excellence: The Symbiosis of AI, DevOps, DataOps and ML Engineering
Achieving commercial excellence also relies on collaboration among DevOps, DataOps, and ML Engineering teams. Working in unison, these cross-functional teams provide the foundation for the seamless execution of commercial strategies. This multidisciplinary approach aligns data, operations, and machine learning, ensuring that AI-driven initiatives sync with commercial objectives while strictly adhering to regulatory compliance and data security standards.
Conclusion
The confluence of MLOps, data, and AI is shaping a new paradigm in pharmaceutical commercial excellence. An array of trends—from personalized customer engagement to real-time dynamic pricing, supply chain optimization, and cross-functional collaboration—herald a future where technology and strategy work together harmoniously.
As the pharmaceutical sector embraces automation, scalability, and democratization, it strides toward a future where well-informed decisions, unwavering regulatory compliance, and streamlined supply chains are the standard. This blending of technical and business excellence will reshape the industry and ultimately yield benefits for patients and healthcare on a global scale.
References
- MLOps: Continuous delivery and automation pipelines in machine learning. Google Cloud. May 18, 2023. Accessed December 12, 2023. https://cloud.google.com/architecture/mlops-continuous-delivery-and-automation-pipelines-in-machine-learning
- Janssen pharmaceuticals increases machine learning accuracy by 21% using Amazon SageMaker. AWS. 2022. Accessed November 22, 2023. https://aws.amazon.com/solutions/case-studies/janssen-case-study/
- Data and AI are helping to get medicines to patients faster. Pfizer Inc. 2022. Accessed November 22, 2023. https://www.pfizer.com/sites/default/files/investors/financial_reports/annual_reports/2022/story/data-and-ai-are-helping-to-get-medicines-to-patients-faster/
- What is AutoML?. AutoML.org. 2023. Accessed November 29, 2023. https://www.automl.org/automl/
- What is edge computing? IBM. Accessed November 29, 2023. https://www.ibm.com/topics/edge-computing
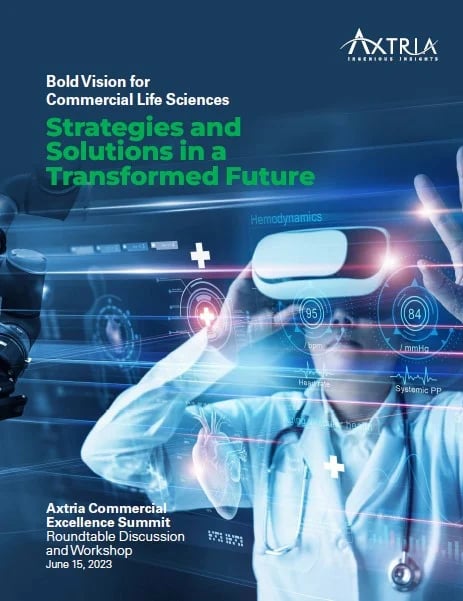
Complete the brief form to download the white paper
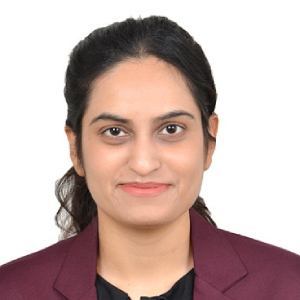
Kaveri Pradeep
Kaveri is a Senior Manager, Data Science at Axtria. She has 10+ years of experience working across the globe with multiple clients across multiple business domains. Having honed her skills at prestigious organizations such as BCG and UHG, she brought her expertise to the cutting-edge environment of Axtria where she currently serves as Senior Manager. Her tenure at these powerhouses has not only broadened her horizons but also equipped her with a unique blend of strategic vision and hands-on experience. At the heart of Kaveri's prowess lies her deep expertise in Machine Learning Engineering and MlOps. As a thought leader, Kaveri has become a driving force behind the seamless integration of machine learning models into the operational fabric of organizations.
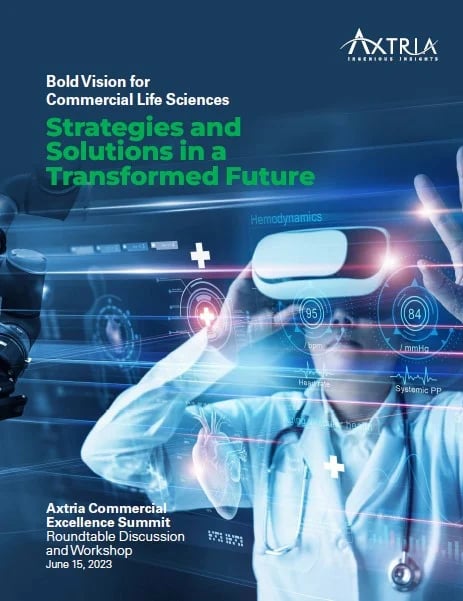
Complete the brief form to download the white paper
Related insights
Stay current on topics you care about
Recommended insights
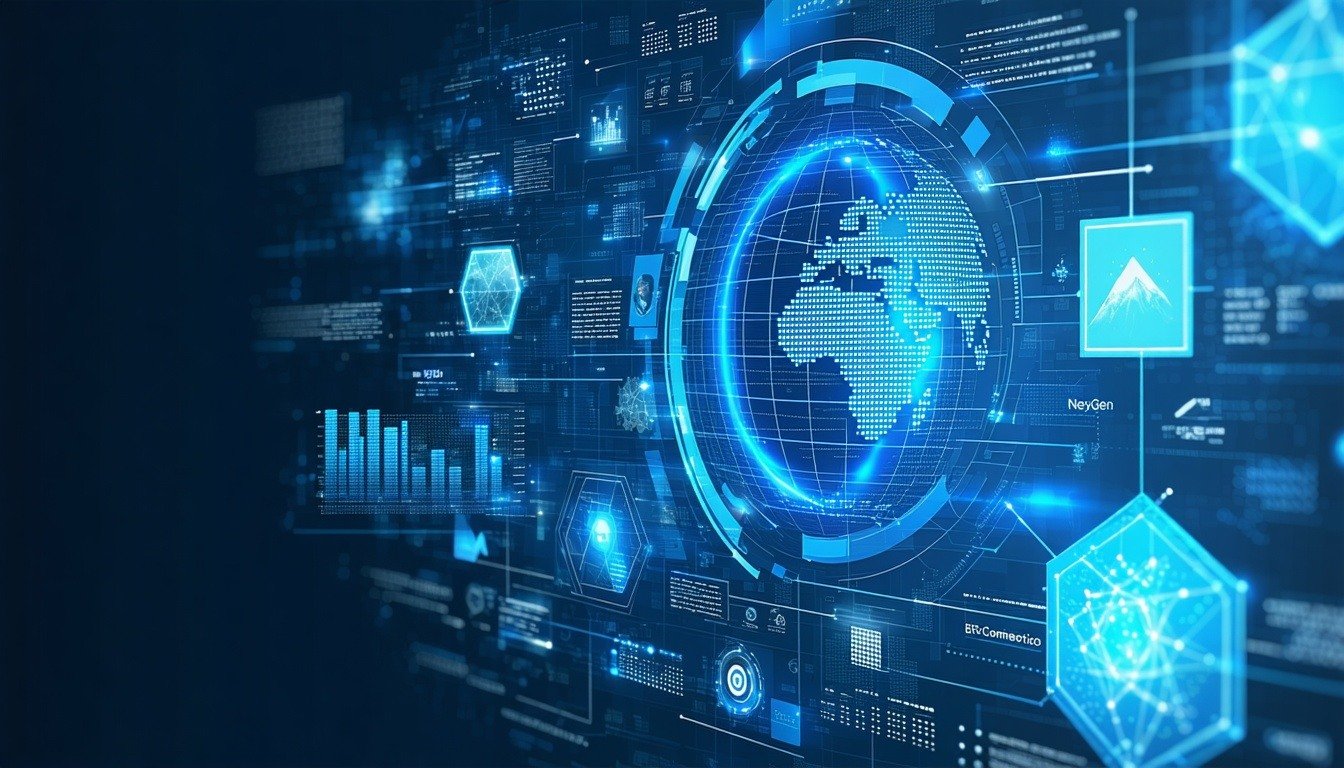
Blog
NexGen Commercial Intelligence: How Generative and Agentic AI are powering Field Force Effectiveness
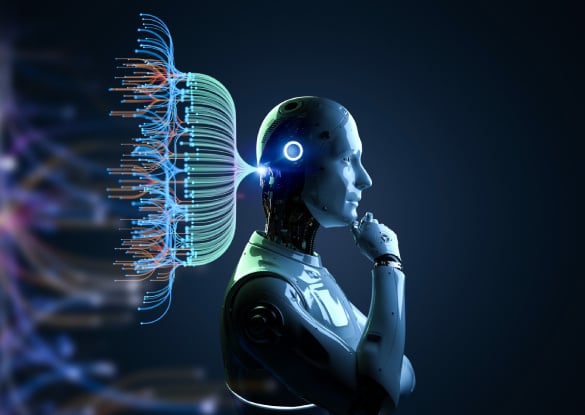
Article