Traditional marketing looks at promotional activities in isolation. For example, a direct-to-consumer (DTC) manager focuses only on DTC content, such as which slots to buy across channels. A sales force (SF) manager focuses on which healthcare professionals (HCPs) to detail and with what frequency. However, looking at the channels in isolation is misleading as this approach ignores the interaction between channels and whether it is complementary or non-complementary. Failure to consider how channels interact may lead to misrepresenting the actual value generated by them. Marketing Mix (MMx) analysis and next best actions (NBAs) can solve this problem.
Marketers and analysts alike often confuse MMx analysis and NBAs
A standard MMx analysis uses multiple promotional channels to inform long-term investment decisions in a classic multi-channel framework, typically over two to three years. The MMx process uses statistical analysis to attribute sales increases to individual promotion channels, allowing the marketer to optimize both medium-term and long-term profits. This process has been prevalent across all industries, including life sciences, and has remained relatively unchanged over the past decade.
On the other hand, NBAs try to optimize returns in the short term by selecting the most appropriate promotional action. The core constructs of an NBA are promotion and message sequences, all of which are optimized using machine learning (ML) models. The current NBA process is primarily based on customers’ engagement with promotional channels.
The data used for both analyses have different granularity levels. NBA models typically use disaggregated data, while MMx relies on data where tactics are aggregated to the channel level. For a truly effective strategy, both need to be intertwined.
Given the differences in data inputs, modeling methodology, planning horizons, and intended use, recommended outputs often contradict one another. For example, MMx could recommend expanding HCP banner advertising, while NBAs could suggest reducing the same activity. Alternately, MMx could mean scaling sales force investment down, while NBA recommendations could imply the opposite. These and similar inconsistencies would lead to questions and issues like:
- Which recommendation should you follow?
- How do you reconcile the recommendations from the two processes?
In this blog, we will outline how the recommendations from MMx and NBA should be adapted to resolve inconsistencies. We will consider the business’ relative weight on long-term vs. short-term, as well as the overall alignment of strategy (MMx) with operations (NBA) for effective customer engagement.
DESCRIBE THE “AS IS” STATE
MMx guides periodic investment decisions by estimating the long-term response of various promotions and optimizing the promotion mix under different budget scenarios. This process entails the following steps:
Strategic recommendations provide high-level guidance for the promotion mix and levels. Examples of typical MMx recommendations might include:
- Increase email activity by 22% over the next 12 months.
- Decrease call frequency by 15% toward Tier 1 and Tier 2 customer segments.
- Prioritize banner ads for critical patient segments.
On the other hand, NBA recommendations are more tactical and are primarily guided by near-term promotions and responses. When implementing a particular marketing strategy, this process suggests the next action for a specific customer based on their response to the most recent promotional activities.
In the NBA process, the first step is to segment customers based on their channel and message affinity, demographics, prescribing patterns, and behavior attributes.
After defining the segments, the ML models look at the most recent data to predict an immediate/short-term outcome likely to be generated from an HCP if exposed to a particular tactic or message. The tactic or message associated with the highest value outcome would then be the recommendation developed for that HCP. Typical examples of NBA recommendations include:
- Make a detail to Dr. Smith and talk about reimbursement benefits.
- Follow up with Dr. Singh with an email and remind him of the product’s superior efficacy.
- Schedule a virtual call with Dr. Jane to address product safety concerns.
Learn More - "Assessing Interaction Among Promotion Channels To Accurately Measure Marketing ROI"
THE PROBLEM
The short-term optimization made through NBAs could contradict the long-term optimization recommendations made by MMx, resulting in significant implications for revenue growth and profitability. These contradictions arise from different time frames and modeling constructs and can be observed by aggregating past NBA recommendations and comparing them with MMx results. NBA recommendations can greatly exceed or significantly fall short of those from MMx, implying that our promotions are no longer optimal (see the figures below).
Source: Axtria Inc. Figure 2: NBA recommendations exceed MMx
Source: Axtria Inc. Figure 3: NBA recommendations fall short of MMx
Let’s explain this further using an example. Here is the typical recommendation from NBA for a particular HCP over one year. The critical thing to note here is the use of Bayesian techniques, which means that the recommendations will vary over time as the NBA model receives new and updated information.
NBA Recommendations | |||
Month | Detailing | Digital | Emails |
1 | 4 | 2 | 4 |
2 | 4 | 2 | 0 |
3 | 3 | 1 | 1 |
4 | 2 | 5 | 3 |
5 | 1 | 4 | 4 |
6 | 2 | 3 | 1 |
7 | 1 | 4 | 4 |
8 | 3 | 4 | 3 |
9 | 4 | 3 | 2 |
10 | 5 | 0 | 2 |
11 | 2 | 1 | 1 |
12 | 5 | 1 | 3 |
Source: Axtria Inc. Table 1: NBA recommendations over one year
The table below shows the aggregated NBA recommendations for one HCP over one year.
NBA Recommendations - Annualized | ||
Detailing | Digital | Emails |
36 | 30 | 28 |
Source: Axtria Inc. Table 2: Annualized NBA recommendations
The following table shows the MMx recommendations for the same HCP for the next one-year period.
MMx Recommendations - Annualized | ||
Detailing | Digital | Emails |
12 | 36 | 24 |
When we compare the two outputs for the same HCP, we notice that while digital and email recommendations are more or less aligned, the detailing recommendations are significantly out of sync.
The question is: "which recommendation should the marketer follow?" If they go with MMx, they are not taking advantage of the local, granular events and insights that emerge from the NBA framework. If the marketer adopts the NBA recommendations, they will not be optimal in the long-term. Also, an HCP getting significantly more promotion because of NBA recommendations may lead to other HCPs not getting enough promotion because of budgetary or capacity constraints.
THE SOLUTION
There are three possible solutions to overcome the problem:
- Use the NBA framework for budgetary assessments. For example, use the NBA models to inform long-term decisions and ignore MMx entirely.
- Use business rules and the MMx models’ outputs to constrain NBA model recommendations.
- Use the outputs of the MMx model as inputs to the NBA model.
The first methodology does not work well because NBA models are based on a short-term horizon where they look at the most recent activity of an HCP to provide near-term recommendations. Using them to make long-term budgetary decisions is inefficient and may be inaccurate.
The second approach is superior to the first because it uses long-term recommendations as guardrails to suggest short-term actions. However, it reduces the efficiency of the NBA by using subjective criteria to influence short-term recommendations.
The third approach uses external business rules to avoid the problem of arbitrarily curtailing short-term recommendations. Instead, the MMx recommendations explicitly pass on to the NBA model as inputs, which influence the construction of the NBA model to yield consistent recommendations. This method uses Bayesian techniques to estimate the NBA model.
Download Case Study - "Axtria Conducted Market Mix Modeling For A Top US Pharma Company"
CONCLUSION
The third option is recommended since it combines both NBA and MMx frameworks without resorting to arbitrary, subjective constraints. However, the analytics involved are complex, expensive, and may not be feasible in all situations. With limited resources, we recommend option two, where some MMx insights could be applied as guardrails to constrain NBA results.
In summary, we should strive for harmonized short- and long-term promotional recommendations for clear direction on promotion priorities, thus maximizing returns on promotional investments.
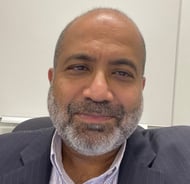
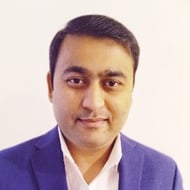