Introduction
It takes 10 to 15 years to develop one successful drug, and the price of new drug approvals has risen to roughly $2.6 BN, according to a study by Tufts Center for the Study of Drug Development.1 Despite these significant investments in time and money, 90% of test drugs that enter clinical trials never get approved.1 Also, clinical development productivity has remained historically low, even though the composite Clinical Development Productivity Index rose in 2020 after declining since 2015, and clinical trial success rates continue to be below average.2 Some clinical trials terminate prematurely, which results in an immense loss of time, money, and human effort. Failure to optimize clinical trial design, inefficient enrollment processes, and poor accrual rates are the main reasons for premature trial closure. According to experts, optimizing the various processes in clinical development is critical for the timely and efficient approval of new products.
This article examines the role of data in optimizing clinical trials, the modern clinical data journey, and how technologies such as predictive analytics, artificial intelligence (AI), and machine learning (ML), when applied to data, can help optimize clinical trial operations and bring cost-efficiency.
Leveraging big data to improve clinical trials operations and outcomes
Clinical trials have evolved to include an ever-expanding array of data sources, increased data volume and precision, decentralized clinical trials, and adaptive designs. Big data plays a significant role in the clinical trial industry, where each trial spans many years, is cost and effort-intensive, and the outcome impacts the wellbeing and lives of people. As clinical trials become increasingly complex, big data offers life sciences companies the opportunity to optimize the clinical trial process by harnessing information from datasets for improved clinical trial design, patient recruitment, site selection, remote patient monitoring, reviewing previous clinical trial events, and even identifying potential side effects before they become a reality.
Intelligent analysis of existing data sources, including biomarkers, genomics, electronic health records (EHRs), claims data, and other types of real-world and clinical trial data, can ultimately lead to improved drug development and faster Food and Drug Administration (FDA) approval. For example, virtual clinical trials are now tapping into patient smartphones through mobile apps and wearable devices. This allows the input of behavioral data sets in real-time. Drug manufacturers can leverage data from wearables and smartphone devices to gain vital insights during their clinical trials. Also, patients’ big data can help life sciences companies consider genetic information as one of the factors to help them identify niche patient populations for more efficient patient recruitment, which ultimately speeds up and reduces the costs of clinical trials.
Further, using real-world data (RWD) to serve as synthetic control arms (SCAs) is modernizing clinical development testing. Synthetic control arms in clinical trials allow avoiding enrolling patients into a trial to receive a placebo against which to compare a legitimate therapeutic treatment. Instead, a synthetic group of the patient population can be created using carefully chosen patients drawn from RWD in the same indication compared to current experimentally treated patients. The data from which this control group is created includes electronic health records (EHRs), administrative claims data, patient-generated data from fitness trackers, home medical equipment, disease registries, and historical trial data. The baseline and disease characteristics are used to select patients to create a historic patient group that matches the experimentally treated group. The data is then used to model or simulate the expected results, comparing them with those in the clinical trial. A synthetic control arm can increase efficiency, reduce delays, reduce trial costs, reduce the risk of late-stage trial failures by providing a better-informed way of go/no-go decision-making, and speed life-saving therapies to market by reducing or eliminating the need to enroll control participants.
With the above backdrop, clinical data management needs to change quickly to keep up with the increased complexity of clinical trials. There is an increasing need to provide real-time visibility into data and clinical trial operations to use it to drive intelligent business processes. The current clinical trial scenario has disrupted the old-school data management methods that life sciences companies followed in the past. Modernized techniques and technologies are being used by clinical data managers as they adapt to the new data management ecosystem in clinical trials.
Learn More - "Optimize Clinical Trial Planning to Drive Trial Success"
Using the modern clinical data journey to optimize clinical trials
Trial data serves as the foundation for the entire drug development cycle. Failure to appropriately organize and manage study data can result in delayed regulatory approvals, leading to extended timelines to reach the market for a promising compound. Data passes through several stages, from collection to storage to processing, and analytics. Following a consistent and structured approach throughout is required.
Deploying modern clinical data management approaches can help ensure drug development programs are future-proof. Clinical organizations are embarking on data and analytics modernization journeys. These journeys need to be planned, keeping in mind the challenges of clinical data and the assessment of current data infrastructure. Life sciences companies are unifying data and workflows on cloud-based platforms aided by advanced analytics and AI to improve data management systems’ scalability, flexibility, intelligence, and interoperability with various third-party systems. A coherent view of several processes helps save time, deal with challenges, and adapt to changes proactively.
Clinical trial software facilitates clinical trials from start to finish, i.e., protocol management, case report form (CRF) design, database design, metadata management, and the collection, analysis, and submission of compliant clinical study data to regulatory authorities. The objective is to accelerate the time-to-market for quality clinical products. The FDA has also encouraged cloud-based clinical trial software solutions to streamline the clinical trial process.
Clinical trial software encompasses several different types of software for different stages of the clinical trial process. These systems provide a rich data set by incorporating day-to-day trial events. Some of them include:
- Clinical Trial Management System (CTMS) is an integrated cloud-based software platform used for the end-to-end management of clinical trials. For example, storing clinical trial project management documents, tracking deadlines and milestones, and centralized billing processes.
- Electronic Data Capture (EDC) is a tool for recording and collecting clinical documents.
- Electronic Clinical Outcome Assessment (eCOA) employs technology such as handheld devices, tablets, or the web allowing patients, clinicians, and caregivers to report outcomes directly, resulting in more granular endpoint data.
- Clinical Data Management System (CDMS is a software used to collect, store, and analyze data from clinical trials conducted at investigator sites.)
Applying Clinical Data Interchange Standards Consortium’s (CDISC is an organization that develops standards and supports data exchange in clinical research) standardization to data from these systems allows for uniformity in clinical documents and helps streamline the trials data. CDISC standardization methods include foundational or data content standards such as Protocol Representation Model (PRM), Clinical Data Acquisition Standards Harmonization (CDASH), Study Data Tabulation Model (SDTM), and Analysis Data Model (ADaM). These are the basic standards for many other CDISC standards that support data collection, management, analysis, and reporting at all stages of the clinical study process. Using CDISC standards is not obligatory, but it is encouraged by regulatory authorities such as the FDA – the submission and review process is usually quicker because all documents (including the Clinical Study Report) are submission-ready at the design stage itself.
Resolving clinical research challenges with predictive analytics
Life sciences companies are increasingly turning to big data and predictive analytics to improve decision-making during the entire clinical trials journey – from protocol approval, country and site selection, study start-up, and patient recruitment to patient retention and database lock and closeout.
Learn More - "Predictive Analytics: What Is It, and Why Is It Critical for Your Business Growth?"
One of the most significant challenges in many clinical trials is meeting enrollment quotas on time to prevent trial termination. According to the Tufts Center for the Study of Drug Development, 37% of sites selected for clinical trial studies under-enroll, and 11% cannot enroll even a single subject.3 Further, while 89% of studies eventually meet enrollment targets, sponsors often meet their targets by doubling their original timelines or engaging in the costly practice of adding rescue studies due to poor enrollment.3 In combination with AI, RWD can improve patient recruitment and make clinical trials more effective and efficient. Big data can show where high concentrations of patients reside, which helps find study sites that can meet recruitment targets on schedule. Focusing on these targeted site prospects can substantially reduce site selection and preparation costs.
Below is an illustration of the questions that can be addressed during the different stages of clinical trials by bringing in a pragmatic approach to designing, conducting, and managing clinical trials using real-world data. To work around these questions, sponsors need to use predictive analytics platforms to help optimize clinical research, anticipate issues, and mitigate some of their problems.
Applying AI and ML to improve clinical trial efficiency
AI and ML tools are transforming how clinical development occurs by accelerating drug discovery and delivering significant cost efficiencies while providing better and faster insights to inform decision-making. AI can be trained to differentiate cancerous cells from normal cells, recognize complex patterns in medical imaging data, and assess large and complex genomic datasets. AI analytics can also combine screening, diagnostic or prognostic information, clinical laboratory results, treatment data, and other information stored in natural language to detect unexpected patterns and predict treatment responses and patient behavior.
ML is a branch of AI in which algorithms and statistical models are used to enable computers to perform specific tasks. ML relies heavily on inference and patterns to address sophisticated challenges. Clinical organizations can use the following ML techniques to determine the relationships between data points and drive decisions:
- Supervised learning algorithms are fed with labeled datasets (i.e., the input/question) and their interpretation (i.e., the output/answer). They learn from the initial, labeled datasets and independently make future decisions and predictions, i.e., answer similar questions from new data.
- Unsupervised learning algorithms are fed with data without their interpretation. This helps find previously unknown patterns in the data set without pre-existing labels.
- Reinforcement learning algorithms learn to react to an environment independently.
Overall, AI/ML is accelerating the ability of life sciences companies to analyze massive data sets and take meaningful action to create a more streamlined research environment.
Learn More - "AI/ML Trends Taking The Life Sciences Industry By Storm"
Conclusion
The future of clinical data management has arrived. It is scalable, flexible, intelligent, and interoperable with third-party tools. This digital transruption – a more sophisticated use of data, tools, technologies, and processes – makes the clinical trial process more efficient. Its patient-focused approach increases the success of clinical trials, thus, accelerating the journey of drugs from the lab to the market shelf, benefitting patients, sponsors, payers, and physicians alike.
Learn More - "The Innovative Path Forward In Clinical Trials"
References
- A Strategy for Making Clinical Trials More Successful. Amgen. Published on October 19, 2018. Available at: https://www.amgen.com/stories/2018/10/a-strategy-for-making-clinical-trials-more-successful
- Global Trends in R&D. IQVIA Institute for Human Data Science. Published in May 2021. Available at: https://www.fdanews.com/ext/resources/files/2021/05-19-21-GlobalTrendsinRD.pdf?1621462621
- Clinical Trials – Why Are Metrics Important in Starting Clinical Trials?. Drug Development and Delivery. Published in January/February 2018. Available at: https://drug-dev.com/clinical-trials-why-are-metrics-important-in-starting-clinical-trials/
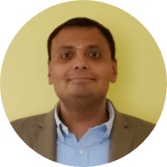
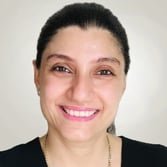